Business Solutions
The Role of NN Models in AI License Plate Recognition
In the rapidly evolving landscape of artificial intelligence, one area garnering significant attention is AI license plate recognition. This technology plays a crucial role in modern vehicle identification systems, offering enhanced accuracy and efficiency. At the heart of this advancement are neural network (NN) models, which have revolutionized how vehicles are tracked and identified. By mimicking the human brain’s processing capabilities, NN models have unlocked new levels of precision in recognizing and interpreting license plate data. As we delve into the role these models play, we’ll explore their transformative impact on AI license plate recognition and how they are shaping the future of automated vehicle monitoring and security systems.

Published
2 months agoon
By
Adva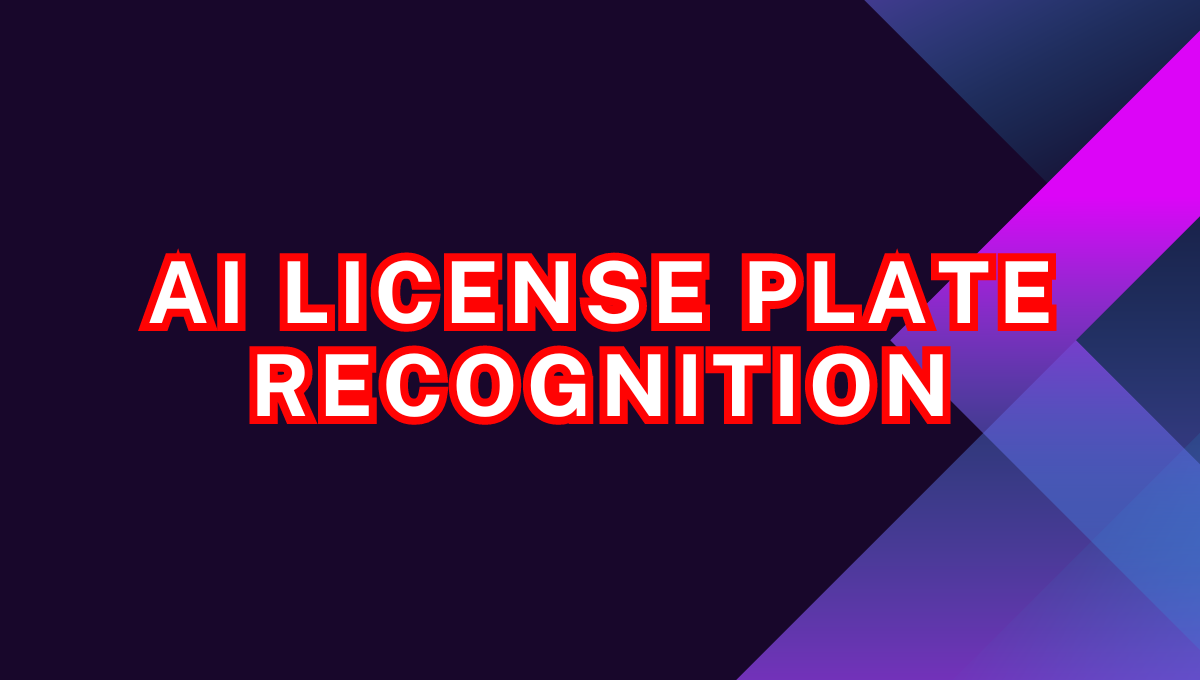
NN models (neural network models) and AI license plate recognition are revolutionizing the way traffic surveillance and law enforcement operate. By integrating advanced neural networks into AI-driven systems, license plate recognition has become faster, more accurate, and capable of real-time decision-making. This combination of NN models and AI license plate recognition offers improved security, more efficient traffic management, and higher accuracy in detecting and identifying vehicles.
Introduction to Neural Network (NN) Models in AI
Neural network models, often referred to as NN models, are a subset of artificial intelligence that mimics the human brain’s ability to recognize patterns, learn from data, and make decisions. These models are built using layers of interconnected neurons that process information in a manner similar to biological neurons. Through deep learning techniques, NN models can analyze large datasets, identify trends, and optimize performance over time.
NN models have gained prominence in AI applications because of their ability to perform complex tasks like image recognition, natural language processing, and predictive analytics. Over the years, neural networks have evolved from simple, shallow models to sophisticated deep neural networks capable of handling intricate real-world scenarios. Their ability to learn autonomously makes them ideal for tasks that require pattern recognition and adaptation, which is precisely why they are now critical in AI license plate recognition systems.
Overview of AI License Plate Recognition Systems
AI license plate recognition is a technology that uses artificial intelligence to automatically capture, identify, and interpret license plate numbers from vehicles in real time. It combines cameras, optical character recognition (OCR), and AI algorithms to detect and read license plates from video or still images. This technology is widely used in traffic management, law enforcement, toll systems, and parking management.
The system typically works by capturing images of vehicles as they pass through designated points like highways, toll booths, or parking lots. The AI-based recognition system then processes these images, extracting the license plate information, and cross-referencing it with databases to identify vehicles involved in criminal activities, violations, or other incidents. AI license plate recognition systems are designed to operate in various lighting conditions, weather environments, and vehicle speeds, making them extremely versatile.
Role of NN Models in License Plate Recognition
The integration of NN models into AI license plate recognition has significantly enhanced the accuracy and speed of these systems. Neural networks are particularly well-suited for this task because they excel at pattern recognition. In license plate recognition, NN models process images of plates and identify letters and numbers, even under challenging conditions, such as poor lighting, occlusion, or low image quality.
By training neural networks on large datasets of license plates, AI systems can learn to recognize a wide range of fonts, plate designs, and conditions. NN models help improve the system’s ability to accurately detect and interpret license plates, even when they are partially obscured or in non-ideal conditions. Additionally, NN models contribute to real-time processing, ensuring that license plates are detected and identified without delays, which is crucial for law enforcement and traffic monitoring.
Improving Pattern Recognition in AI License Plate Systems
Neural networks play a key role in improving pattern recognition in AI license plate systems, particularly through optical character recognition (OCR). OCR is the technology that converts the visual representation of license plates into machine-readable text. However, recognizing characters from images is not always straightforward. Factors such as font variation, distortion, blurriness, and dirt on the plates can impact recognition accuracy.
NN models enhance OCR’s effectiveness by continuously learning and improving their ability to recognize different fonts, sizes, and license plate formats. Through deep learning, these models can adjust to variations in character shapes, handle degraded images, and identify plates even when they are partially damaged. For instance, when a license plate is dirty or covered by dust, traditional OCR systems may fail to read the plate accurately. In contrast, NN models can adapt to such situations, using learned data patterns to accurately recognize the plate numbers.
Benefits of Using NN Models in AI License Plate Recognition
One of the main benefits of using NN models in AI license plate recognition is the significant boost in accuracy and speed. Traditional systems often struggle with inconsistent lighting, moving vehicles, and varying angles of license plates. NN models, however, can handle these complexities due to their ability to learn from large datasets and improve their recognition algorithms over time.
The ability to process data in real time is another major benefit. NN models enable AI license plate recognition systems to identify vehicles and provide actionable information within seconds, which is critical in high-pressure situations like law enforcement operations. The enhanced precision provided by NN models helps reduce false positives and missed detections, resulting in more reliable data for authorities.
Another advantage of NN models is their scalability. As cities grow and traffic volumes increase, the demand for more robust license plate recognition systems also rises. Neural networks can scale efficiently, allowing them to handle larger datasets and process higher volumes of license plate detections without compromising performance.
Training NN Models for License Plate Recognition
Training neural network models for license plate recognition requires extensive data collection and preprocessing. Large datasets of images containing various types of license plates are necessary to train the neural network. These datasets include plates from different regions, countries, and environmental conditions, ensuring that the model is versatile and accurate.
The training process involves feeding the neural network thousands of labeled images, where the correct characters and numbers of each license plate are identified. The neural network adjusts its internal parameters to reduce recognition errors and improve performance. Deep learning techniques are particularly effective in this stage, allowing the model to learn complex features of license plates, such as variations in fonts, spacing, and image distortions.
Regular updates and retraining are required to maintain the system’s performance as new vehicle license plate designs or formats are introduced. Neural networks also benefit from fine-tuning, where specific adjustments are made to optimize performance for different regions or use cases.
Challenges of Implementing NN Models in AI License Plate Recognition
While NN models bring substantial advantages, implementing them in AI license plate recognition systems presents several challenges. One of the key challenges is dealing with diverse environmental factors such as varying lighting conditions, weather, and vehicle speeds. Neural networks need to be trained extensively to handle these conditions effectively, which requires large and diverse datasets.
Another challenge is hardware and software integration. Neural networks can be computationally intensive, requiring powerful processors and optimized software to function in real time. Ensuring that these systems operate efficiently on existing hardware infrastructures, such as surveillance cameras and traffic systems, can be complex and costly.
Additionally, balancing performance with data privacy is a growing concern. License plate recognition systems must comply with data protection regulations, such as GDPR, to ensure that the information collected is stored and used responsibly.
Enhancing Security with AI License Plate Recognition
AI license plate recognition is a valuable tool for enhancing security in cities, on highways, and in private sectors. With the ability to identify vehicles involved in criminal activities, AI-based systems can alert authorities in real time when a suspicious vehicle is detected. By integrating NN models into these systems, the recognition process becomes faster and more accurate, making it easier to catch criminals or monitor high-risk areas.
For law enforcement agencies, AI license plate recognition provides critical support in identifying stolen vehicles, tracking suspects, and enforcing traffic violations. NN models allow these systems to function 24/7, monitoring vehicle activity and flagging potential threats immediately. This not only improves public safety but also streamlines law enforcement efforts.
Future Trends in NN Models for License Plate Recognition
As AI and neural network technology continue to advance, the future of NN models in license plate recognition holds tremendous potential. One of the key trends is the development of more sophisticated deep learning models capable of handling increasingly complex recognition tasks. With advances in AI, neural networks will be able to process higher-resolution images, handle more challenging environmental factors, and improve real-time processing speeds.
Additionally, AI advancements will lead to more autonomous and integrated systems. Future license plate recognition technologies may incorporate other AI capabilities, such as predictive analytics, to anticipate traffic patterns or detect suspicious vehicle behavior. The integration of 5G networks and IoT (Internet of Things) technology will further enhance the connectivity and responsiveness of these systems.
Best Practices for Deploying AI License Plate Recognition Systems
When deploying AI license plate recognition systems powered by NN models, it is essential to follow best practices to ensure optimal performance. First, gathering comprehensive and diverse datasets for training is crucial. The quality and variety of the data will directly impact the system’s ability to recognize license plates accurately in different conditions.
Second, optimizing NN models for specific scenarios is important. Whether the system is used in a high-traffic urban environment or a more controlled private facility, adjusting the neural network’s parameters can significantly improve its accuracy and speed. Regular updates and retraining should also be conducted to keep the system up to date with new license plate designs or formats.
Lastly, ensuring that the system complies with data privacy laws and regulations is critical. Implementing data encryption, access controls, and secure storage practices will help protect sensitive vehicle and personal information.
FAQs
- What are NN models in AI?
NN models, or neural network models, are a subset of artificial intelligence that mimics the human brain’s neural structures to process data, recognize patterns, and make decisions. They are used in complex tasks such as image recognition and predictive analytics. - How does AI license plate recognition work?
AI license plate recognition uses cameras and artificial intelligence algorithms, including optical character recognition (OCR), to detect and read license plates from images or video footage in real time. It identifies and interprets license plate numbers for various applications like traffic management and law enforcement. - What role do NN models play in license plate recognition?
NN models enhance the accuracy and speed of AI license plate recognition by improving the system’s ability to detect patterns and recognize numbers and letters, even in challenging conditions such as poor lighting or obscured plates. - How do NN models improve pattern recognition in AI license plate systems?
NN models improve pattern recognition by continuously learning from large datasets. They help optical character recognition (OCR) systems handle diverse fonts, sizes, and damaged or obscured license plates, increasing recognition accuracy. - What are the benefits of using NN models in AI license plate recognition?
The primary benefits include high accuracy, real-time processing, scalability, and reduced errors in detecting and identifying license plates. NN models allow the system to function efficiently under various environmental conditions.
As a freelance tech and startup news writer, I'm always looking to stay up-to-date with the latest in the industry. I have a background in web development and marketing, so I'm particularly interested in how new startups are using technology to change the world.
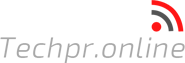
You may like
Business Solutions
Why RF Products Matter in RF Over Glass Technology
Published
2 days agoon
November 25, 2024By
Roze Ashley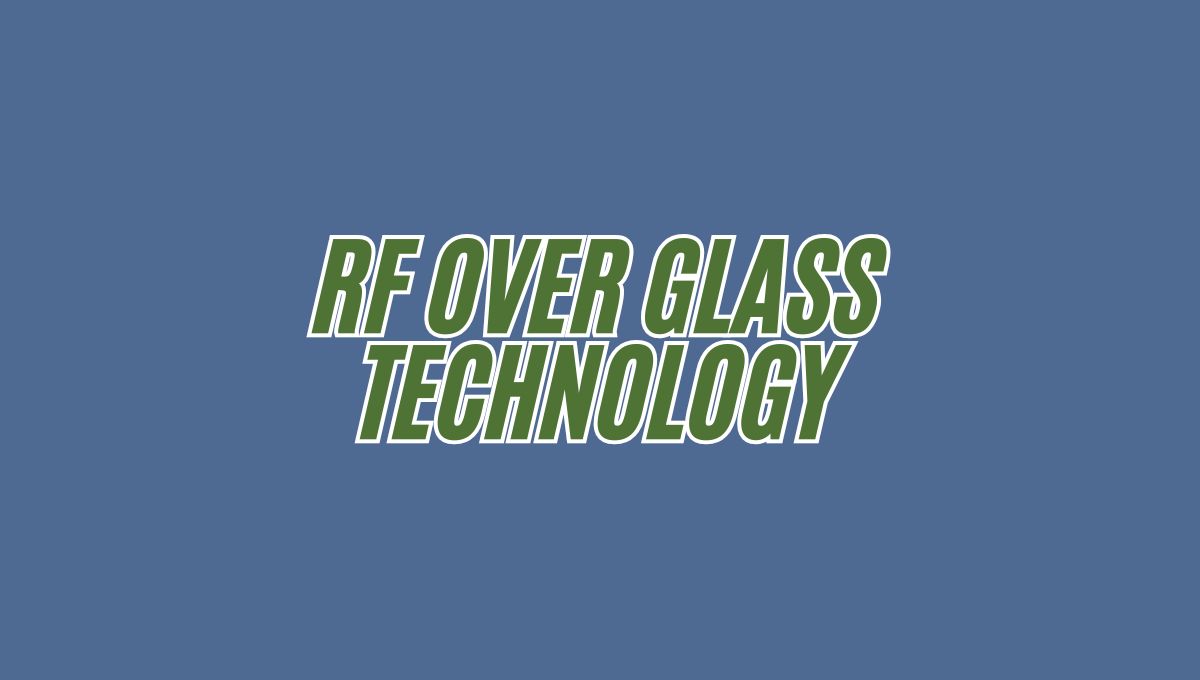
RF over Glass and RF products are redefining what’s possible in modern communication networks, offering solutions that combine the best of RF and fiber optic technologies. With their ability to deliver high-quality, interference-free signals over long distances, RFoG systems are particularly valuable for network operators looking to upgrade their infrastructure and meet rising demands for bandwidth.
By providing scalable, reliable, and high-speed connectivity, RFoG technology and RF products support various industries, from telecommunications and broadcasting to smart cities and IoT. While challenges like installation costs and technical requirements exist, the long-term benefits make RFoG a worthwhile investment for future-ready networks. As technology advances, RFoG and RF products will continue to drive innovation in connectivity, powering the networks that keep us all connected.
How RF Over Glass Works in Communication Systems
RF over Glass, or RFoG, operates by converting traditional radio frequency signals for transmission over fiber optic cables, combining the benefits of RF technology with the advantages of fiber optics. Unlike standard coaxial cables that are prone to interference and signal loss, fiber optic cables in RFoG systems transmit signals as light pulses, allowing for greater distances without compromising quality.
RFoG uses a system of optical transmitters and receivers to convert and manage RF signals. On the transmitting end, an RF signal is converted into a light signal by an optical transmitter. This light signal is then sent through fiber optic cables to the receiver, where it’s converted back into an RF signal. This process enables clear, interference-free communication and supports high-bandwidth applications, making RFoG ideal for modern broadband and telecommunication networks.
This technology also simplifies network upgrades for operators transitioning from coaxial systems to fiber optic networks. RFoG systems are compatible with existing RF equipment, allowing service providers to enhance their infrastructure without completely overhauling their setup. This flexibility makes RFoG a cost-effective solution for networks in need of higher performance and bandwidth.
Advantages of RF Over Glass for Network Operators
RFoG offers multiple advantages over traditional RF systems, particularly for network operators aiming to improve service quality and scalability. One of the primary benefits of RFoG is its ability to provide clearer signals over long distances. Fiber optic cables are less susceptible to interference from electromagnetic sources, meaning RFoG systems can maintain high signal integrity even in areas with significant electronic noise.
Another major advantage of RFoG is its scalability. Fiber optic networks can handle large amounts of data, making RFoG ideal for supporting future bandwidth demands. As network usage grows and more devices connect to the internet, the need for high-capacity systems becomes increasingly important. RFoG allows providers to scale their networks efficiently without sacrificing performance, ensuring they can meet the connectivity needs of modern consumers.
RFoG also reduces the need for amplifiers and other intermediate equipment typically required in coaxial cable systems. By minimizing these components, RFoG networks are more energy-efficient and cost-effective in the long term, making it a practical choice for large-scale deployments.
Essential RF Products for RFoG Implementation
Implementing RFoG requires a variety of RF products that enable signal conversion, amplification, and transmission. Key components in RFoG setups include RF converters, amplifiers, and transceivers, each playing a critical role in maintaining signal quality and ensuring compatibility across the network.
RF converters are vital in RFoG systems as they convert RF signals to optical signals and vice versa. This conversion process is essential for integrating RF technology with fiber optic infrastructure. Amplifiers, although used less frequently in RFoG compared to coaxial systems, help boost signal strength in specific applications or environments with challenging terrain.
Transceivers are another important RF product in RFoG setups. These devices send and receive signals, allowing two-way communication over fiber optic networks. High-quality transceivers ensure that data transmission remains reliable, supporting real-time applications like video streaming, gaming, and teleconferencing.
Together, these RF products enable RFoG technology to deliver fast, reliable, and high-quality communication, making it a robust solution for network operators looking to upgrade their infrastructure.
Improving Signal Quality with RFoG and RF Products
One of the significant benefits of RFoG and its related RF products is the improved signal quality it brings to communication networks. Traditional RF systems often struggle with issues like signal degradation and interference, especially over long distances or in urban environments with high electromagnetic activity. RFoG addresses these challenges by transmitting signals through fiber optic cables, which are immune to electromagnetic interference.
With the help of RF products such as converters and amplifiers, RFoG systems can maintain a strong and clear signal throughout the network. Amplifiers can be strategically placed to enhance signal strength when needed, while RF converters ensure that signals are transmitted in their optimal format. This combination of RFoG technology and RF products creates a stable and high-quality communication environment, essential for applications where clarity and consistency are critical.
Furthermore, RFoG’s ability to support higher frequencies without degradation makes it well-suited for advanced applications, such as high-definition video streaming and large-scale data transfers. This capacity to handle high-frequency signals without losing quality enables service providers to offer superior services to their customers.
RF Over Glass vs. Traditional RF Systems
When comparing RF over Glass to traditional RF systems, several key differences emerge, particularly in terms of performance, scalability, and cost-effectiveness. Traditional RF systems rely on coaxial cables, which can experience significant signal loss over long distances and are prone to interference from external sources. RFoG, on the other hand, uses fiber optic cables that provide high fidelity and are less affected by environmental interference.
In terms of scalability, RFoG outperforms traditional RF systems. As the demand for bandwidth continues to grow, coaxial cables may struggle to keep up without extensive upgrades. Fiber optic cables used in RFoG systems can support much larger amounts of data, making them more future-proof than coaxial cables.
While the initial cost of installing RFoG may be higher due to fiber optic infrastructure requirements, the long-term benefits and reduced need for maintenance can make it more cost-effective. The choice between RFoG and traditional RF depends on the specific needs of the network, but for operators seeking to deliver high-speed, reliable service, RFoG offers distinct advantages.
Key Applications of RF Products in Different Industries
RF products, including those used in RFoG systems, are essential across various industries. In telecommunications, RF products enable the delivery of high-speed internet and mobile data services. RF converters and amplifiers support consistent, high-quality signals for data and voice communications, ensuring that users have a seamless experience.
The broadcasting industry also benefits significantly from RF products, as they support signal clarity for television and radio transmissions. RF amplifiers and converters are crucial for transmitting high-quality audio and visual data, providing a reliable source of information and entertainment to viewers.
In emerging fields such as smart cities and IoT, RF products facilitate the smooth transmission of data from connected devices, supporting applications such as remote monitoring, traffic management, and public safety. RFoG technology is particularly valuable here, as it enables large-scale data transmission over fiber networks, providing the infrastructure needed for IoT devices to communicate effectively.
Challenges in Deploying RF Over Glass Networks
While RFoG offers numerous benefits, deploying this technology can pose several challenges. One of the primary obstacles is the cost of building a fiber optic infrastructure, which requires significant investment upfront. Additionally, some regions may lack the existing infrastructure necessary to support RFoG, necessitating more extensive installations.
Compatibility with legacy systems is another concern. While RFoG is compatible with many RF products, integrating it into older networks can be complex, requiring specialized equipment and technical expertise. Network operators must plan carefully to ensure a smooth transition, as well as consider ongoing maintenance and operational costs associated with fiber optics.
Technical expertise is also essential, as RFoG systems require knowledgeable staff for installation, maintenance, and troubleshooting. Finding skilled technicians can be challenging, especially for operators transitioning from traditional coaxial systems to fiber optics.
Future Trends in RF Over Glass and RF Products
The future of RFoG and RF products is filled with exciting advancements, driven by the growing demand for high-speed, reliable communication. As fiber optic technology continues to evolve, RFoG is expected to become more accessible and efficient, with improvements in data capacity, speed, and reliability. These developments will support the expansion of next-generation networks, including 5G and beyond.
AI integration is another trend in RF technology, enabling automated network management and optimizing data transmission. AI algorithms can analyze network traffic in real-time, making adjustments to frequencies and signal strengths for optimal performance. This will enhance RFoG’s capabilities and make RF products even more adaptable and efficient.
Miniaturization of RF products is also expected to grow, making it possible to integrate RF technology into smaller devices and applications, such as wearable devices and portable communication systems. These trends point to a future where RFoG and RF products will play an even more significant role in delivering seamless, high-quality connectivity.
FAQs on RF Over Glass Products
- What is RF over Glass (RFoG), and how does it work?
RFoG is a technology that transmits RF signals over fiber optic cables, converting radio frequencies into light for clearer and long-distance communication. - How does RFoG improve signal quality?
RFoG reduces interference and signal loss, providing higher clarity and reliability compared to traditional coaxial systems. - What are the main benefits of RFoG for network operators?
RFoG offers clearer signals, scalability, reduced need for amplifiers, and compatibility with existing RF infrastructure, making it ideal for modern networks. - What RF products are essential for RFoG systems?
Key RF products include RF converters, amplifiers, and transceivers, which support signal conversion, boosting, and two-way communication in RFoG systems. - How do RF converters work in RFoG setups?
RF converters change RF signals to optical signals and vice versa, enabling integration with fiber optic networks for efficient data transmission. - How does RFoG compare to traditional RF systems?
Unlike traditional coaxial systems, RFoG uses fiber optics, which offer less interference, better scalability, and superior signal quality over long distances. - In which industries are RF products commonly used?
RF products are widely used in telecommunications, broadcasting, defense, and smart cities, supporting reliable communication and data transmission. - What challenges do operators face when implementing RFoG?
Challenges include high installation costs, compatibility issues with legacy systems, and the need for specialized technical expertise. - What future advancements are expected for RFoG technology?
Advancements in data capacity, AI integration, and miniaturization are expected, making RFoG more efficient and adaptable to new applications. - How does RFoG benefit modern communication networks?
RFoG enhances connectivity by delivering high-speed, interference-free data transmission, supporting the growing demand for reliable and scalable networks.
Business Solutions
The Importance of Industrial Ethernet Switches in IIoT
Published
2 days agoon
November 25, 2024By
Roze Ashley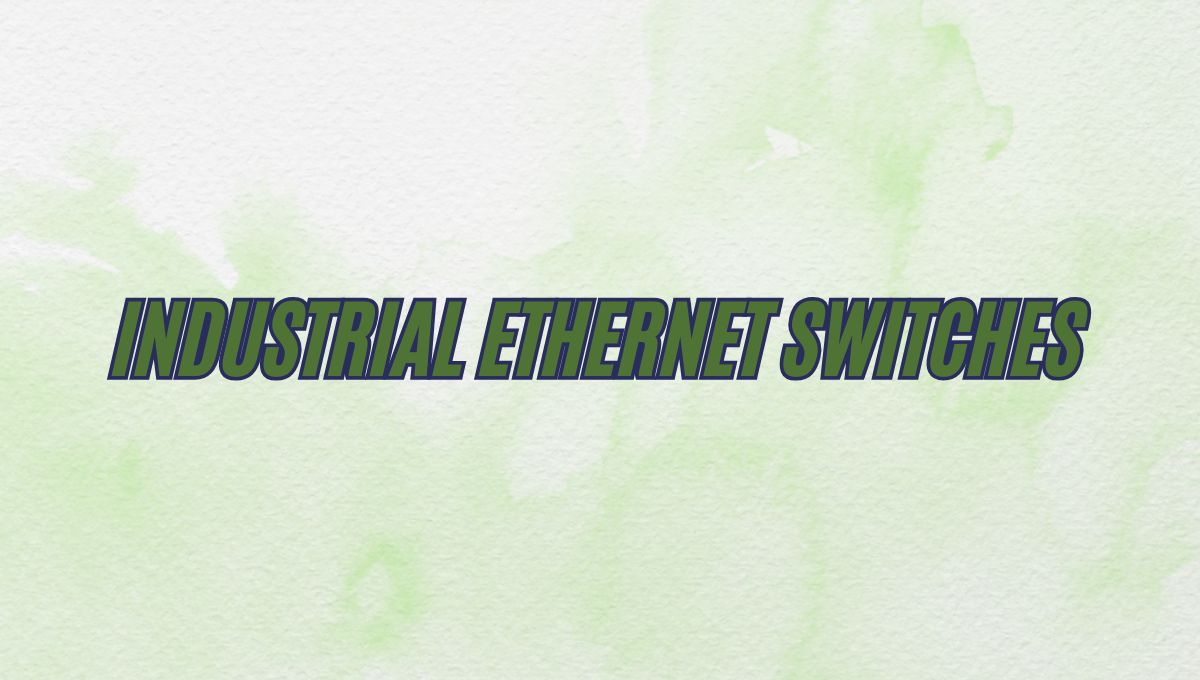
Industrial Ethernet switches and IIoT solutions are revolutionizing industrial operations by enabling seamless connectivity, advanced data processing, and real-time monitoring across diverse industrial environments.
Industrial Ethernet switches play a crucial role in modern IIoT solutions by providing reliable connectivity for devices and systems used in manufacturing, logistics, and energy sectors. Unlike standard Ethernet switches used in office environments, industrial Ethernet switches are designed to withstand harsh industrial conditions, including extreme temperatures, vibration, and electromagnetic interference. These devices ensure that IIoT networks remain stable and secure, even in environments with high levels of dust, humidity, or other challenging conditions.
The advent of Industry 4.0 has increased the need for resilient and secure networks to support connected devices, sensors, and automated equipment across vast industrial spaces. Industrial Ethernet switches fulfill this requirement, enabling high-speed data transmission and uninterrupted connectivity, essential for real-time monitoring and data-driven decision-making in complex IIoT networks.
What Makes an Ethernet Switch “Industrial”?
An Ethernet switch is classified as “industrial” based on its ability to perform reliably under challenging conditions, which can include physical durability, temperature tolerance, and long-term dependability. Standard Ethernet switches typically operate within limited temperature ranges and may be susceptible to dust, moisture, and other contaminants. Industrial Ethernet switches, however, are built with ruggedized materials, allowing them to endure extreme temperatures, ranging from -40°C to 75°C, as well as exposure to chemicals and electromagnetic interference.
These switches often include additional features such as redundancy protocols, specialized ports, and extended power capabilities. For example, many industrial Ethernet switches support Power over Ethernet (PoE), enabling devices to receive both power and data over the same connection. This is especially useful in industrial environments where deploying separate power sources for each device would be costly or impractical.
Understanding IIoT Solutions and Their Applications
IIoT, or the Industrial Internet of Things, refers to the network of connected devices, machines, and systems used to collect, analyze, and act on data within industrial settings. IIoT solutions are designed to optimize operations, improve safety, and reduce costs by enabling more informed, data-driven decisions. IIoT solutions find applications across various industries, including manufacturing, energy, logistics, and agriculture, where remote monitoring, automation, and predictive maintenance are crucial.
In manufacturing, IIoT solutions enable equipment to communicate seamlessly with other systems on the factory floor, allowing for automated processes, real-time monitoring, and timely equipment maintenance. In the energy sector, IIoT can improve grid reliability by monitoring substations and adjusting energy distribution based on demand. Similarly, in logistics, IIoT solutions enhance supply chain visibility by tracking goods in real time and optimizing transportation routes for efficiency.
Building Robust IIoT Networks with Industrial Ethernet Switches
For IIoT solutions to function effectively, they require a network infrastructure capable of supporting large volumes of data with minimal latency. Industrial Ethernet switches are integral to these networks, as they manage data transmission between IIoT devices and central processing systems. By providing high-speed connections and redundancy protocols, these switches ensure that IIoT networks remain operational even if certain parts of the network are disrupted.
A robust IIoT network depends on fast and reliable data transmission to support real-time monitoring and analysis. Industrial Ethernet switches allow data to be processed close to the source, reducing latency and improving overall network performance. Redundant connections and backup power options also contribute to network resilience, making it possible to maintain operations without interruptions even in case of device or connection failures.
Key Features of Industrial Ethernet Switches for IIoT
Industrial Ethernet switches offer several key features that make them ideal for IIoT applications. One of the primary features is Power over Ethernet (PoE), which enables devices to receive power directly through the Ethernet cable, eliminating the need for separate power sources. PoE simplifies installation, reduces infrastructure costs, and makes it easier to expand IIoT networks as new devices are added.
Another essential feature is network redundancy, which allows switches to establish multiple connections for critical devices. This redundancy ensures continuous data flow even if a connection fails, providing higher reliability in mission-critical applications. Additionally, industrial Ethernet switches often support managed protocols, allowing network administrators to monitor network traffic, configure settings remotely, and troubleshoot issues.
IIoT-Driven Benefits in Modern Industrial Environments
The integration of IIoT solutions into industrial environments offers numerous benefits, from operational efficiency to enhanced safety. IIoT-driven automation allows industrial systems to respond quickly to changes in production demands, helping companies streamline processes and improve productivity. In addition, real-time data collection and analysis enable predictive maintenance, where equipment issues are detected early to prevent costly breakdowns and reduce downtime.
Safety is another significant benefit, as IIoT-enabled sensors and monitoring systems can detect hazardous conditions and trigger alerts before accidents occur. For instance, in industries where employees work in dangerous environments, IIoT can monitor factors like air quality, temperature, and equipment status, alerting operators if conditions become unsafe. With IIoT solutions, companies can create safer work environments while improving operational efficiency.
Comparing Industrial Ethernet Switch Types for IIoT
Industrial Ethernet switches come in various types, each suited to different network configurations and IIoT needs. Managed switches, for instance, offer advanced capabilities such as remote configuration, monitoring, and network segmentation. These features make managed switches ideal for large, complex IIoT networks requiring detailed control and monitoring.
Unmanaged switches, on the other hand, are simpler and require minimal setup, making them suitable for smaller IIoT networks where extensive control isn’t necessary. Meanwhile, Power over Ethernet (PoE) switches provide the added advantage of powering devices directly through Ethernet connections, which is particularly beneficial in environments with limited access to power sources.
Selecting the right type of industrial Ethernet switch depends on the specific needs of the IIoT network, the size of the operation, and the level of control required for efficient management.
Overcoming Challenges in IIoT Network Deployment
Deploying IIoT solutions with industrial Ethernet switches presents certain challenges, including compatibility issues, data security, and network scalability. Many industrial environments feature legacy systems that may not easily integrate with modern IIoT technologies. Ensuring compatibility between new and existing devices can require custom solutions and specialized expertise.
Data security is another critical challenge. As IIoT networks grow, they become attractive targets for cyber threats. Industrial Ethernet switches must be equipped with security protocols to protect data integrity and prevent unauthorized access. Additionally, scalability is essential for companies looking to expand their IIoT networks over time. A flexible network infrastructure with modular switch configurations helps companies grow their IIoT capabilities without major overhauls.
Best Practices for Implementing IIoT with Industrial Ethernet Switches
Implementing IIoT solutions with industrial Ethernet switches requires strategic planning and careful execution. One best practice is to prioritize network segmentation, which involves dividing the network into smaller sections. This approach limits the potential impact of a cyberattack or system failure, as isolated segments are easier to secure and manage.
Regular maintenance and monitoring are also crucial for maintaining a stable IIoT network. Industrial Ethernet switches should be routinely checked for performance issues, software updates, and hardware integrity. Finally, companies should implement cybersecurity measures tailored to industrial environments, such as encryption, multi-factor authentication, and firewall protection, to prevent unauthorized access and ensure data privacy.
FAQs on Industrial Ethernet Switches and IIoT Solutions
- What role do industrial Ethernet switches play in IIoT solutions?
Industrial Ethernet switches provide the backbone for IIoT networks, enabling secure, high-speed data transmission essential for real-time monitoring and automation in industrial environments. - How do industrial Ethernet switches differ from regular Ethernet switches?
Industrial Ethernet switches are built to withstand harsh conditions, such as extreme temperatures, dust, and vibration, making them ideal for demanding industrial settings. - What are the key applications of IIoT solutions in industry?
IIoT solutions are widely used in manufacturing, logistics, energy, and other sectors for tasks like remote monitoring, predictive maintenance, and automation, improving overall efficiency and safety. - How do industrial Ethernet switches contribute to reliable IIoT networks?
These switches support redundancy protocols and high-speed data processing, ensuring network stability and uninterrupted operation, even in cases of partial network failures. - What are essential features of industrial Ethernet switches for IIoT?
Key features include Power over Ethernet (PoE), network redundancy, real-time monitoring, and remote management, which are crucial for maintaining reliable IIoT networks. - How do IIoT solutions improve productivity in industrial settings?
IIoT enables automation and real-time data analysis, which reduces downtime, improves safety, and supports better decision-making, leading to more efficient operations. - What types of industrial Ethernet switches are used in IIoT applications?
There are various types, such as managed, unmanaged, and PoE switches, each offering different levels of control, flexibility, and functionality for different IIoT requirements. - What challenges are commonly faced when implementing IIoT with Ethernet switches?
Challenges include ensuring compatibility with legacy systems, maintaining data security, and scaling the network to support future expansion, all of which require careful planning.
Business Solutions
Understanding Satellite and Space Cameras
Published
2 days agoon
November 25, 2024By
Roze Ashley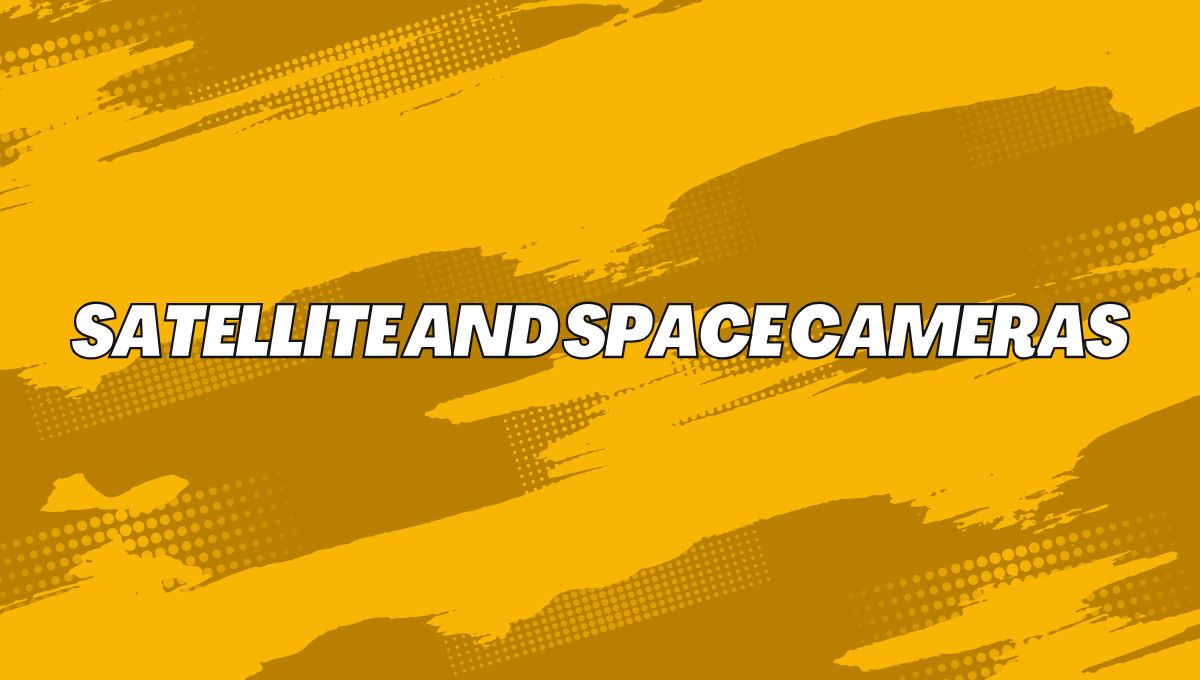
Looking to the future, satellite cameras are set to become even more powerful and versatile. Emerging technologies like quantum sensors could enable cameras to capture even more detailed images at a fraction of today’s size. Artificial intelligence will likely continue to improve image processing, making it possible to identify features and patterns instantly.
In the next decade, as humanity prepares for missions to the Moon, Mars, and beyond, the demand for high-quality space cameras will only grow. Space cameras on these missions will not only document these journeys but also analyze planetary surfaces, atmospheric conditions, and even search for signs of life. Here on Earth, satellite cameras will continue to play an essential role in monitoring the environment, supporting agriculture, and enhancing global security, paving the way for a more connected and informed world.
Different Types of Satellite and Space Cameras
Satellite cameras come in various forms, each specialized for unique tasks. Among the most fascinating are multispectral and hyperspectral cameras, which capture images across multiple wavelengths of light. Multispectral cameras, for instance, can “see” light that is invisible to the human eye, such as infrared, making them perfect for monitoring vegetation health or water quality. Hyperspectral cameras take this concept even further, capturing hundreds of narrow spectral bands, which can reveal detailed chemical compositions of rocks, soils, and atmospheres.
Thermal cameras capture temperature differences, helping scientists monitor volcanic activity, wildfires, or urban heat islands. For those seeking breathtaking detail, panchromatic cameras provide ultra-high resolution, grayscale images. Each type of camera serves as a unique lens through which we can analyze our world—and beyond.
Why Resolution Matters in Space Imaging
When it comes to satellite cameras, resolution is everything. Imagine viewing your neighborhood from space with a camera that can distinguish features just one meter apart—such a camera could easily spot a parked car or a building’s outline. Resolution in satellite imaging is measured in meters per pixel; the lower the number, the sharper the image.
Achieving this level of detail, however, is no small feat. A satellite camera’s resolution depends on factors like lens quality, sensor sensitivity, and distance from Earth. Recent technological advancements have made it possible to capture imagery with astonishing clarity, but increasing resolution also poses challenges. More data requires more bandwidth and storage, demanding innovative solutions in data compression and transmission to deliver those crisp, high-definition images to researchers around the world.
Cutting-Edge Advancements in Satellite Camera Technology
Advances in satellite camera technology are pushing boundaries that seemed impossible just a few years ago. Artificial intelligence (AI) is now playing a major role, enhancing the way images are processed and analyzed. For example, AI can automatically identify features in an image, such as forests, rivers, or urban areas, reducing the workload for human analysts.
Meanwhile, miniaturization is another exciting trend. With the advent of CubeSats—small, lightweight satellites that can be launched in constellations—satellite imaging is no longer limited to a handful of large, costly satellites. Instead, dozens or even hundreds of small satellites, each equipped with its own camera, can now monitor Earth’s surface, offering frequent updates and richer datasets than ever before. These advancements promise a future where even the most remote parts of the Earth are constantly within view.
Watching Over Nature: Satellite Cameras in Environmental Monitoring
Satellite cameras play an indispensable role in environmental science. From tracking deforestation in the Amazon to monitoring ice melt in the Arctic, these cameras give scientists a bird’s-eye view of critical environmental changes. By capturing images over time, satellite cameras help reveal trends that would otherwise go unnoticed, such as the gradual shrinking of glaciers or the spread of desertification.
One key area of environmental monitoring is climate change. For instance, satellites track greenhouse gas emissions and measure sea levels, providing data that helps scientists predict future climate scenarios. Additionally, satellite cameras monitor natural disasters in real-time, allowing governments and aid organizations to respond quickly to events like hurricanes, floods, and wildfires. With every image, satellite cameras provide valuable insights into the health of our planet.
A Silent Guardian: Satellite Cameras in Security and Defense
In security and defense, satellite cameras offer a silent yet vigilant presence. Military and government agencies around the world rely on these cameras to monitor borders, track potential threats, and gather intelligence on critical sites. High-resolution images can reveal details such as troop movements, equipment placements, and infrastructure changes, offering a strategic advantage to those who can see from above.
Satellite cameras used in defense are often equipped with additional capabilities, such as radar imaging, which allows them to “see” through clouds or darkness. This means that monitoring can continue day or night, in any weather. With these eyes in the sky, nations can respond more effectively to threats and better protect their citizens, maintaining peace and security on a global scale.
From Sky to Soil: Satellite Cameras in Agriculture and Land Management
Farmers and land managers are increasingly turning to satellite cameras as a tool for optimizing agriculture. Satellite imagery allows farmers to monitor crop health, assess soil quality, and track water usage, all from above. By using detailed data, they can target areas that need attention, resulting in increased yields and reduced waste.
For instance, satellite cameras can detect early signs of crop disease or water stress, helping farmers take preventive action before problems escalate. In addition, land managers use satellite imagery to track deforestation, soil erosion, and land use changes. This “bird’s-eye” perspective makes it easier to implement sustainable practices and make informed decisions about land conservation.
Frequently Asked Questions
- What are satellite cameras and space cameras?
Satellite cameras and space cameras are specialized imaging devices used on satellites and space probes to capture images of Earth and outer space, providing valuable data for research, environmental monitoring, and security. - How do satellite cameras capture images from space?
Satellite cameras use a combination of advanced optics, sensors, and data relays to gather light, convert it into digital data, and transmit the images back to Earth for analysis. - What are the different types of satellite and space cameras?
There are various types, including multispectral, hyperspectral, thermal, and panchromatic cameras, each capturing different wavelengths and suited for specific applications like environmental monitoring and high-resolution imaging. - Why is resolution important in satellite imaging?
Resolution determines the clarity and detail of satellite images, which is crucial for tasks like urban planning, environmental monitoring, and defense operations. - What recent advancements have been made in satellite camera technology?
Recent advancements include AI-enhanced imaging, miniaturization of components, and the development of CubeSats, all of which improve image quality, frequency of data capture, and overall efficiency. - How do satellite cameras contribute to environmental monitoring?
Satellite cameras monitor environmental changes, track climate impacts, and help manage natural resources by providing data on issues like deforestation, glacier melt, and natural disasters. - How are satellite cameras used in security and defense?
These cameras are vital for surveillance, border monitoring, and intelligence gathering, providing high-resolution images that can reveal strategic information like troop movements and infrastructure changes. - What role do satellite cameras play in agriculture?
Satellite cameras aid agriculture by monitoring crop health, assessing soil moisture, and improving resource management, helping farmers optimize crop yields and reduce environmental impact. - What challenges exist in developing high-performance satellite cameras?
Challenges include designing cameras that can withstand space’s harsh conditions, managing data storage and transmission, and creating compact, high-resolution systems suitable for satellite payloads. - What is the future of satellite and space camera technology?
Future advancements may include quantum sensors, enhanced AI processing, and cameras capable of supporting deep-space exploration, with applications expanding in both space missions and Earth observation.

Why RF Products Matter in RF Over Glass Technology

The Importance of Industrial Ethernet Switches in IIoT

Understanding Satellite and Space Cameras
Trending
-
Marketing & Analytics2 years ago
A Complete Guide To HubSpot’s New B2B Marketing, Sales Hub, and Prospecting Tool
-
3D Technology1 year ago
3D Scanner Technology for Android Phones: Unleashing New Possibilities
-
Marketing & Analytics2 years ago
How SMS Services And Software For Bulk SMS Sending Can Help Your Business Grow
-
3D Technology2 years ago
Mobile 3D Scanners: Revolutionizing 3D Scanning Technology
-
3D Technology2 years ago
3D scanning technologies and scanning process
-
Business Solutions11 months ago
Understanding A2P Messaging and the Bulk SMS Business Landscape
-
Business Solutions10 months ago
The Power of Smarts SMS and Single Platform Chat Messaging
-
Automotive1 year ago
DSRC vs. CV2X: A Comprehensive Comparison of V2X Communication Technologies