Tech
Operational Technology Monitoring (OT) Power and Smart Diagnostics
Welcome to the future of industrial operations! In a world driven by technological advancements, it’s crucial for businesses to stay ahead of the game. That’s where Operational Technology Monitoring (OT) Power and Smart Diagnostics come into play, revolutionizing the way industries operate. Whether you’re in manufacturing, energy production, or any other field reliant on machinery and systems, this blog post will delve into the fascinating world of OT monitoring and its powerful impact on operational efficiency. So fasten your seatbelts as we take you through an exhilarating journey uncovering how OT Power and Smart Diagnostics are reshaping industries worldwide!

Published
1 year agoon
By
Adva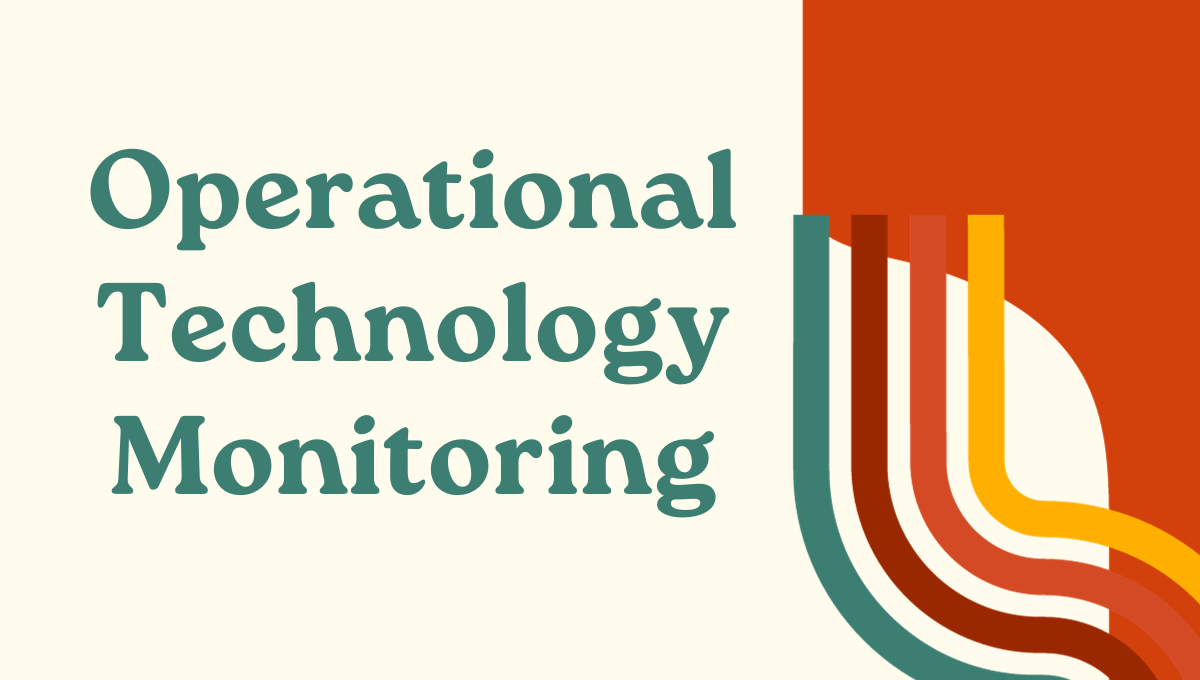
Businesses and industries rely heavily on operational technology (OT) to drive efficiency, productivity, and innovation. OT encompasses the hardware and software used to monitor and control physical processes, making it a critical component of various sectors, from manufacturing to energy production. To stay competitive and resilient, organizations are turning to OT monitoring and smart diagnostics to optimize their operations.
The Essence of OT Monitoring
OT monitoring is a vital discipline that involves the continuous observation and analysis of OT systems and processes. It provides real-time insights into the health and performance of industrial systems and enables organizations to make informed decisions. Here are some key aspects of OT monitoring:
- Real-Time Data Collection: OT monitoring solutions collect data from sensors, devices, and industrial equipment in real-time. This data includes information on temperature, pressure, flow rates, energy consumption, and more.
- Performance Analysis: The collected data is analyzed to assess the performance of industrial processes. It helps identify anomalies, inefficiencies, and potential issues that can impact operations.
- Predictive Maintenance: One of the primary benefits of OT monitoring is its ability to predict equipment failures. By analyzing historical data and patterns, organizations can schedule maintenance proactively, reducing downtime and costly repairs.
- Efficiency Optimization: OT monitoring helps organizations optimize energy usage, streamline production processes, and enhance resource allocation. This leads to cost savings and improved overall efficiency.
- Enhanced Safety: Monitoring critical processes and equipment ensures a safer working environment. Any deviations from safety standards can trigger alarms and immediate corrective actions.
The Rise of Smart Diagnostics
Smart diagnostics is a game-changer in the world of operational technology. It leverages advanced technologies, such as artificial intelligence (AI) and machine learning (ML), to provide real-time insights and actionable recommendations. Here’s how smart diagnostics is making a difference:
- Data Analytics and AI: Smart diagnostics systems use AI algorithms to analyze vast amounts of data quickly. This enables them to detect patterns, anomalies, and trends that may not be apparent through traditional methods.
- Proactive Issue Identification: By continuously analyzing data, smart diagnostics can identify potential issues before they lead to system failures. This proactive approach minimizes downtime and production disruptions.
- Condition-Based Monitoring: Smart diagnostics systems employ condition-based monitoring, allowing organizations to assess the health of equipment and processes in real-time. This data-driven approach improves decision-making and resource allocation.
- Predictive Analytics: Predictive analytics, a subset of smart diagnostics, forecasts future outcomes based on historical data. This capability is invaluable for maintenance planning and resource optimization.
- Remote Monitoring and Control: Smart diagnostics often include remote monitoring and control capabilities, enabling organizations to oversee operations from anywhere. This is particularly beneficial for industries with distributed assets.
Impacts Across Industries
The adoption of OT monitoring and smart diagnostics is reshaping various industries, enhancing their competitiveness and sustainability. Here are some examples of how these technologies are making an impact:
- Manufacturing: In manufacturing, OT monitoring and smart diagnostics optimize production lines, reduce downtime, and improve product quality. Predictive maintenance ensures that machines are serviced precisely when needed, minimizing disruptions.
- Energy: In the energy sector, smart diagnostics help manage and optimize power generation and distribution. This leads to increased energy efficiency, reduced costs, and a more reliable energy supply.
- Healthcare: In healthcare, OT monitoring plays a critical role in ensuring the reliability and availability of medical equipment. Smart diagnostics enhance patient care by minimizing equipment failures and downtime.
- Agriculture: In agriculture, these technologies are used to monitor and manage irrigation systems, weather conditions, and crop health. This data-driven approach maximizes crop yields while conserving resources.
- Transportation: The transportation industry benefits from OT monitoring and smart diagnostics by ensuring the safety and reliability of vehicles, aircraft, and infrastructure. Predictive maintenance prevents breakdowns and accidents.
Challenges and Considerations
While OT monitoring and smart diagnostics offer significant advantages, organizations must address some challenges:
- Data Security: With the increased collection and analysis of data, security and privacy concerns become paramount. Implementing robust cybersecurity measures is essential.
- Integration Complexity: Integrating OT monitoring and smart diagnostics solutions with existing infrastructure can be complex. Organizations must plan for seamless integration to avoid disruptions.
- Skill Gaps: Leveraging these technologies may require specialized skills and training. Organizations should invest in employee development to harness their full potential.
- Scalability: As organizations grow, the scalability of OT monitoring and smart diagnostics solutions becomes critical. Ensuring that systems can handle expanding data volumes and complexity is vital.
Operational technology (OT) is the backbone of industries across the board. The convergence of OT monitoring and smart diagnostics is ushering in a new era of efficiency, safety, and competitiveness. Organizations that embrace these technologies gain a strategic advantage by minimizing downtime, reducing operational costs, and enhancing overall performance.
In a world where data is king, OT monitoring and smart diagnostics empower organizations to make data-driven decisions, proactively address issues, and optimize processes. This leads to greater resilience and agility, ensuring that businesses can thrive in an ever-changing landscape. As industries continue to evolve, the power of these technologies will only become more apparent, driving innovation and progress across sectors.
OT Monitoring and Smart Diagnostics Frequently Asked Questions
Q1: What is OT monitoring, and why is it essential for industrial processes? A1: OT monitoring involves continuous data collection and analysis of operational technology systems and processes. It is crucial because it provides real-time insights into the health and performance of industrial operations, allowing for proactive decision-making and issue resolution.
Q2: What are the primary benefits of predictive maintenance enabled by OT monitoring? A2: Predictive maintenance, a key benefit of OT monitoring, helps organizations reduce downtime and costly repairs by identifying equipment failures before they occur. This proactive approach leads to improved operational efficiency and cost savings.
Q3: How does smart diagnostics differ from traditional monitoring methods in industrial settings? A3: Smart diagnostics leverages advanced technologies like artificial intelligence (AI) and machine learning (ML) to analyze data quickly and accurately. It can detect patterns and anomalies that may not be evident through traditional monitoring methods, enabling more proactive and precise decision-making.
Q4: What industries can benefit from the adoption of smart diagnostics and OT monitoring? A4: Smart diagnostics and OT monitoring have wide-ranging applications across various industries, including manufacturing, energy, healthcare, agriculture, and transportation, among others. These technologies offer efficiency improvements and cost savings in diverse operational settings.
Q5: How do organizations ensure data security when implementing OT monitoring and smart diagnostics? A5: Data security is paramount when implementing these technologies. Organizations should implement robust cybersecurity measures, including encryption, access controls, and regular security audits, to protect sensitive data collected during monitoring and diagnostics.
Q6: What challenges might organizations face when integrating OT monitoring and smart diagnostics solutions into their existing infrastructure? A6: Integration complexity can be a challenge, especially when connecting new monitoring and diagnostics systems with legacy infrastructure. Organizations must carefully plan and execute integration to avoid disruptions and ensure compatibility.
Q7: How can organizations address skill gaps when adopting smart diagnostics and OT monitoring technologies? A7: Addressing skill gaps often involves investing in employee development and training programs. Organizations can also consider partnering with technology providers that offer support and expertise in implementing these technologies.
Q8: Are there specific scalability considerations when implementing OT monitoring and smart diagnostics for growing organizations? A8: Scalability is crucial for growing organizations. They should ensure that their chosen solutions can handle increasing data volumes and complexity as the organization expands. Scalability planning should be an integral part of the implementation process.
Q9: Can remote monitoring and control capabilities be integrated into OT monitoring and smart diagnostics systems? A9: Yes, many smart diagnostics and OT monitoring solutions offer remote monitoring and control capabilities. This enables organizations to oversee and manage operations from anywhere, providing flexibility and convenience.
Q10: What is the future outlook for OT monitoring and smart diagnostics in industrial settings? A10: The future of these technologies is promising, with advancements in AI, ML, and data analytics expected to enhance their capabilities further. As industries continue to embrace digital transformation, the role of OT monitoring and smart diagnostics will become increasingly critical in optimizing operations and ensuring competitiveness.
As a freelance tech and startup news writer, I'm always looking to stay up-to-date with the latest in the industry. I have a background in web development and marketing, so I'm particularly interested in how new startups are using technology to change the world.
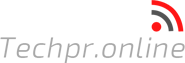
You may like
Tech
The Speed of Sight: Low-Latency HEVC for Military Situational Awareness
Transmitting high-quality video in military operations is no small feat, especially in high-stakes environments where every second counts. Enter low-latency HEVC, a technology transforming how real-time video is delivered and processed on the battlefield. By compressing video efficiently without sacrificing clarity, HEVC ensures seamless situational awareness, empowering faster decision-making and improved mission coordination. This breakthrough is critical for modern military strategies, where accurate and instant information can mean the difference between success and failure. Discover how HEVC is redefining operational effectiveness.

Published
6 days agoon
March 21, 2025By
Adva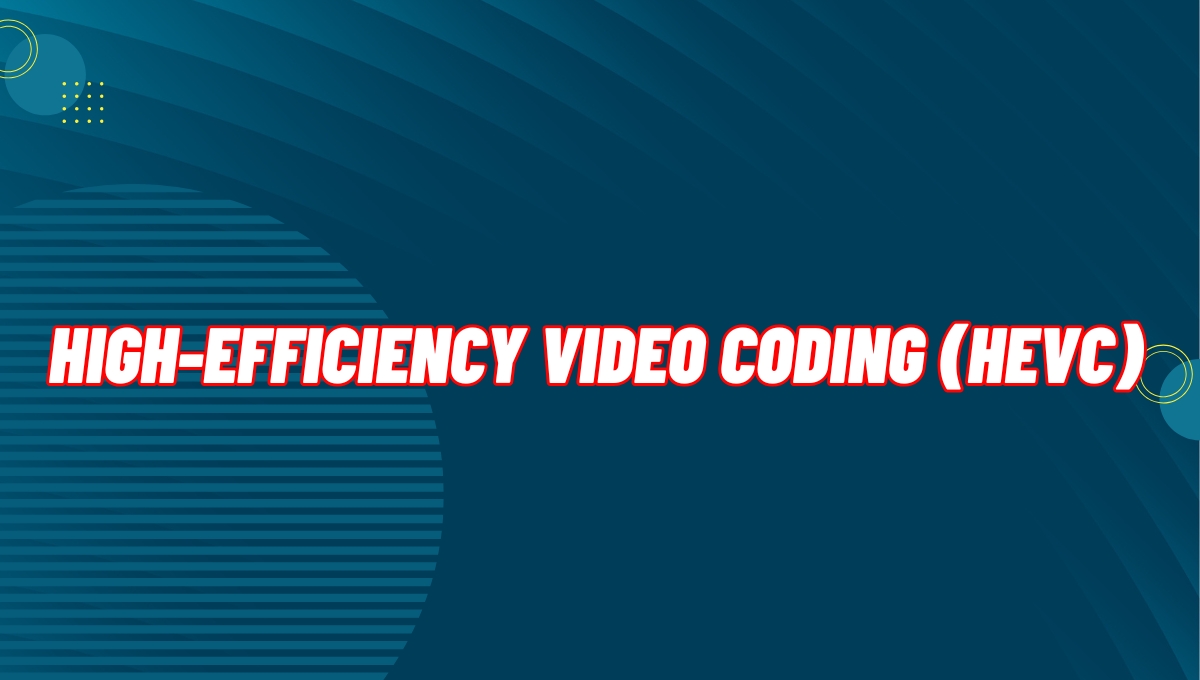
In the high-stakes theater of modern warfare, information dominance hinges on the ability to rapidly acquire, process, and disseminate critical intelligence. Real-time video feeds from drones, surveillance platforms, and soldier-worn cameras are indispensable tools, but their effectiveness is inherently tied to the latency of video encoding and decoding. This is where low-latency High Efficiency Video Coding (HEVC) encoders and decoders are proving transformative for military situational awareness.
The Latency Challenge in Military Video Transmission
Military operations often demand immediate access to visual information. Delays in video transmission can lead to critical misinterpretations, missed opportunities, and compromised safety. Traditional video encoding and decoding methods, particularly those relying on cloud-based processing, introduce significant latency due to network congestion and processing overhead.
HEVC and Low-Latency Encoding: A Game Changer
HEVC, also known as H.265, offers superior compression efficiency compared to its predecessors, allowing for higher-quality video transmission at lower bitrates. However, achieving low latency with HEVC requires specialized encoding and decoding techniques.
Optimized Encoding Parameters:
By carefully adjusting encoding parameters, such as GOP (Group of Pictures) structure, frame rate, and quantization parameters, encoders can minimize processing delays and reduce latency.
Slice-Based Encoding and Decoding: Dividing video frames into smaller slices allows for parallel processing, reducing encoding and decoding times.
Predictive Encoding and Decoding Techniques:
Advanced predictive algorithms can anticipate future frames, reducing the amount of data that needs to be transmitted and processed.
Hardware Acceleration:
Utilizing dedicated hardware accelerators, such as GPUs and specialized ASICs, can significantly speed up HEVC encoding and decoding.
Military Situational Awareness Applications:
Real-Time Drone Surveillance:
Low-latency HEVC enables commanders to receive near-instantaneous video feeds from drones, providing tactical situational awareness for tactical decision-making.
Soldier-Worn Camera Systems:
Real-time video feeds from soldier-worn cameras enhance situational awareness for ground troops, enabling rapid threat assessment and improved coordination.
Remote Battlefield Monitoring:
Low-latency HEVC allows for remote monitoring of critical infrastructure and strategic locations, providing early warning of potential threats.
Target Acquisition and Tracking:
Real-time video feeds from surveillance platforms enable rapid target acquisition and tracking, improving the accuracy of weapon systems.
Command and Control (C2) Systems:
Low-latency HEVC integrates seamlessly with C2 systems, providing commanders with a comprehensive and up-to-date view of the battlefield.
Remote Medical Assistance:
In situations where a medic cannot be on site, a medical professional can view a live low latency feed, and provide real time instructions for the treatment of wounded soldiers.
Benefits for Military Operations:
Enhanced Real-Time Situational Awareness:
Low-latency HEVC provides commanders with near-instantaneous access to critical visual information.
Improved Decision-Making:
Reduced latency enables faster and more accurate decision-making in dynamic and time-sensitive situations.
Increased Operational Effectiveness:
Real-time video feeds enhance coordination, improve target acquisition, and reduce response times.
Enhanced Soldier Safety:
Near real time feeds help to prevent ambushes, and other dangers.
The Future of Low-Latency HEVC in Military Applications:
As military technology continues to evolve, the demand for low-latency video transmission will only increase. Future developments will focus on:
Further reducing latency through advanced encoding and decoding techniques.
Developing more robust and resilient video transmission systems for harsh environments.
Integrating AI-powered video analytics with low-latency HEVC to enhance situational awareness.
Improving the bandwidth efficiency of HEVC to enable transmission over limited networks.
Low-latency HEVC encoders and decoders are revolutionizing military situational awareness, providing commanders with a decisive advantage in the modern battlespace. By enabling real-time access to critical visual information, these technologies are playing a vital role in ensuring the safety and effectiveness of military operations.
Business Solutions
Luxury Real Estate in Ra’anana, Israel
Discover the allure of Luxury Real Estate in Ra’anana, Israel, where modern elegance meets serene suburban charm. Renowned for its upscale homes, beautifully landscaped neighborhoods, and vibrant community, Ra’anana offers a premier lifestyle. Explore sophisticated villas and exclusive residences in one of Israel’s most sought-after locations, perfect for refined living.

Published
7 days agoon
March 20, 2025By
Marks Strand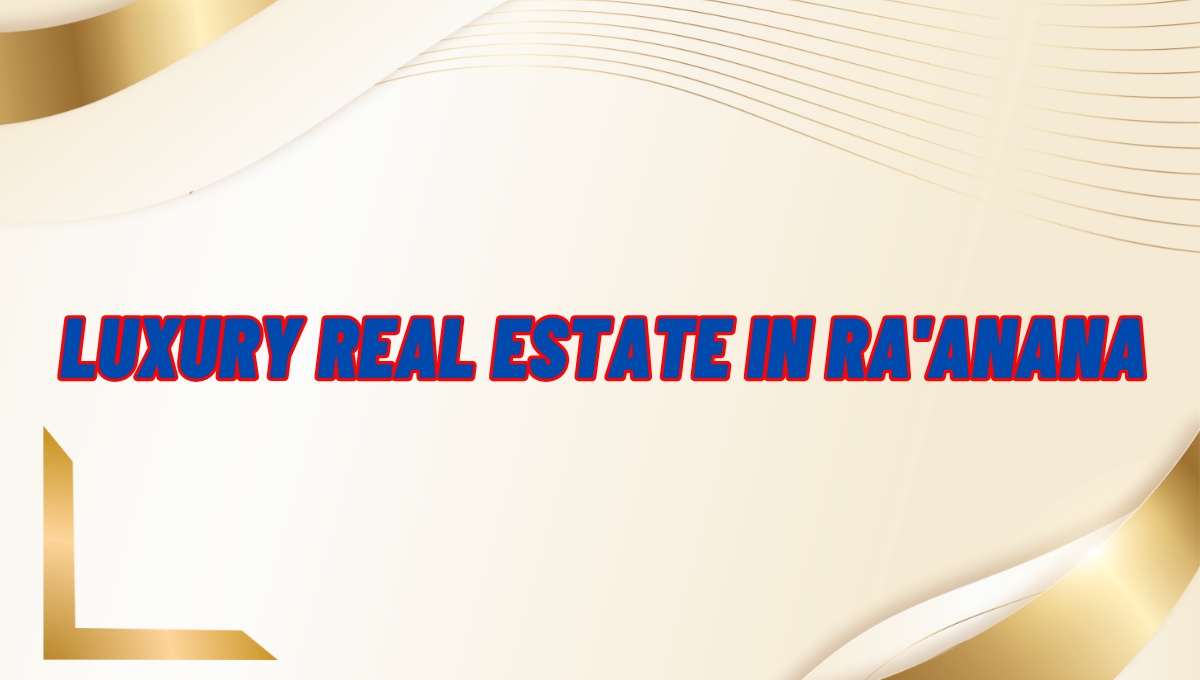
Raanana: A Haven for Luxury Living in Israel
Raanana, a charming city nestled just north of Tel Aviv, has become a haven for luxury living in Israel. Renowned for its upscale atmosphere, lush greenery, and excellent quality of life, Raanana attracts discerning homebuyers seeking a sophisticated and tranquil environment.
The Raanana Luxury Real Estate Market
The Raanana luxury real estate market is characterized by a diverse range of properties, from modern villas and spacious apartments to historic mansions and exclusive gated communities. These properties often feature high-end finishes, private gardens, swimming pools, and breathtaking views.
Popular Locations for Luxury Real Estate in Raanana
Several areas in Raanana have emerged as hotspots for luxury real estate buyers:
- Neve Zemer: This prestigious neighborhood is home to some of the most sought-after properties in Raanana, featuring large villas, private gardens, and a tranquil atmosphere.
- The City Center: The heart of Raanana offers a vibrant mix of modern high-rises and renovated historic buildings, providing a cosmopolitan lifestyle with easy access to amenities and entertainment.
- The Green Areas: Raanana boasts numerous parks and green spaces, and properties located near these areas offer a serene and peaceful living environment.
Key Considerations for Luxury Home Buyers in Raanana
If you are considering purchasing luxury real estate in Raanana, there are several factors to keep in mind:
- Budget: The cost of luxury real estate in Raanana can vary significantly depending on the location, size, and amenities of the property.
- Lifestyle: Consider your lifestyle and how it aligns with the location and amenities of the property. For example, if you are looking for a family-friendly neighborhood, Neve Zemer might be a good option.
- Investment Potential: Raanana is a stable and growing city, making it a sound investment for luxury real estate buyers.
The Appeal of Raanana
Raanana’s appeal stems from several factors:
- High Quality of Life: Raanana offers excellent schools, a wide range of cultural and recreational activities, and a safe and welcoming community.
- Proximity to Tel Aviv: Raanana is conveniently located just a short drive from Tel Aviv, providing easy access to the city’s vibrant cultural and business scene.
- Green Spaces and Parks: Raanana boasts numerous parks and green spaces, offering residents a tranquil and relaxing environment.
Raanana offers a unique and sophisticated lifestyle for luxury homebuyers in Israel. With its diverse range of properties, excellent amenities, and tranquil atmosphere, Raanana is an ideal choice for those seeking a high-quality living experience.
Additional Resources:
Business Solutions
Shielding the Modern Fleet: Robust Vehicle Cybersecurity Strategies in the Age of Connectivity
In the age of smart vehicles and constant connectivity, cybersecurity has become a critical concern for fleet managers and car manufacturers alike. Modern vehicles are packed with advanced technology, making them both efficient and vulnerable to cyber threats. This blog dives into the importance of robust vehicle cybersecurity, exploring cutting-edge strategies to safeguard connected fleets against potential risks. Whether you oversee commercial fleets or personal vehicles, understanding these strategies is key to staying one step ahead of evolving cyber threats. Stay secure, stay connected, and take control of your vehicle’s safety!

Published
1 week agoon
March 19, 2025By
Adva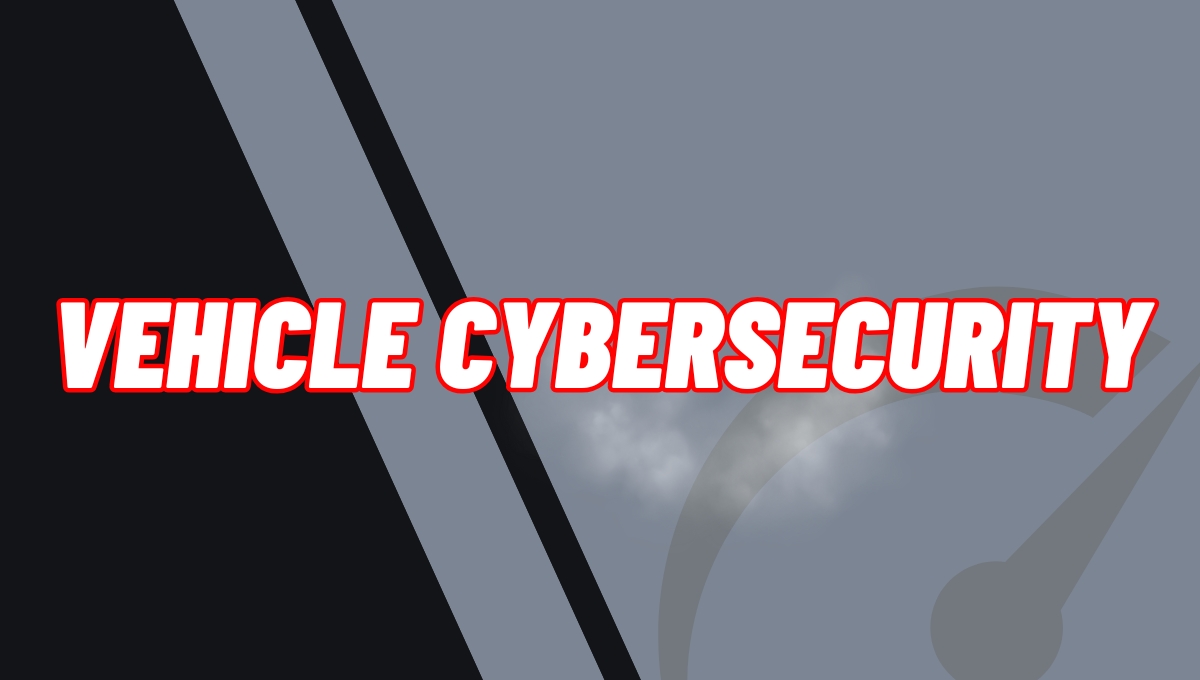
The modern vehicle, a marvel of interconnected systems, is increasingly vulnerable to cyber threats. As cars transform into rolling computers, a comprehensive cybersecurity strategy is no longer optional—it’s imperative. OEMs must adopt a multi-layered approach to protect vehicles from evolving threats, encompassing everything from secure design principles to automated threat detection in the automotive cybersecurity sector.
Building Security In: The Foundation of a Robust Strategy
The first line of defense lies in embedding security into the vehicle’s architecture from the ground up. This involves:
Secure Design Principles: Implementing security by design, ensuring that security considerations are integrated into every stage of the development lifecycle. This includes threat modeling, vulnerability assessments, and secure coding practices.
Hardware Security Modules (HSMs): Utilizing dedicated hardware modules to protect sensitive cryptographic keys and perform secure operations. This provides a secure foundation for authentication, encryption, and other security functions.
Secure Communication Protocols: Employing robust communication protocols, such as encrypted CAN bus communication and secure Ethernet, to protect data transmitted within the vehicle’s network.
Secure Boot and Firmware Updates: Implementing secure boot mechanisms to ensure that ECUs boot from trusted firmware and utilizing secure over-the-air (OTA) update capabilities to patch vulnerabilities and deploy security enhancements.
Automated Threat Detection: TARA Cybersecurity and Beyond
As the complexity of vehicle systems increases, manual threat analysis becomes impractical. Automated threat detection, particularly through Threat Analysis and Risk Assessment (TARA) for cybersecurity, is essential for proactive defense.
TARA Cybersecurity:
This methodology involves systematically identifying and assessing potential threats to the vehicle’s cybersecurity.
It uses automated tools to analyze system architectures, identify potential attack vectors, and assess the impact of potential attacks.
TARA enables manufacturers to prioritize security measures and allocate resources effectively.
This process should be continuously repeated throughout the vehicles life cycle, as new threats emerge.
Intrusion Detection and Prevention Systems (IDPS):
Deploying IDPS solutions that monitor vehicle networks for anomalies and suspicious activity.
These systems can use machine learning algorithms to detect subtle threats that traditional signature-based systems might miss.
Security Information and Event Management (SIEM):
Centralizing security logs and events from various vehicle systems to provide a comprehensive view of the vehicle’s security posture.
SIEM systems can correlate events and identify patterns that indicate potential attacks.
Vulnerability Scanning and Penetration Testing:
Regularly conducting automated vulnerability scans and penetration tests to identify and address security weaknesses.
This proactive approach helps to discover vulnerabilities before malicious actors can exploit them.
Beyond the Vehicle: A Holistic Approach
A comprehensive cybersecurity strategy extends beyond the vehicle itself, encompassing the entire ecosystem:
Supply Chain Security: Ensuring that all components and software used in the vehicle are sourced from trusted suppliers and undergo rigorous security testing.
Cloud Security: Protecting data transmitted to and from the cloud, as well as securing cloud-based services used by the vehicle.
Incident Response: Developing a robust incident response plan to handle cybersecurity incidents effectively, including containment, eradication, and recovery.
Collaboration and Information Sharing: Fostering collaboration and information sharing among manufacturers, suppliers, and cybersecurity experts to stay ahead of evolving threats.
Vehicle cybersecurity is an ongoing process that requires a multi-faceted approach. By combining secure design principles, automated threat detection, and a holistic security strategy, manufacturers can help to protect vehicles from cyberattacks and ensure the safety and security of drivers and passengers in the connected age.

The Speed of Sight: Low-Latency HEVC for Military Situational Awareness

Luxury Real Estate in Ra’anana, Israel

Shielding the Modern Fleet: Robust Vehicle Cybersecurity Strategies in the Age of Connectivity
Trending
-
Marketing & Analytics2 years ago
A Complete Guide To HubSpot’s New B2B Marketing, Sales Hub, and Prospecting Tool
-
3D Technology2 years ago
3D Scanner Technology for Android Phones: Unleashing New Possibilities
-
Marketing & Analytics2 years ago
How SMS Services And Software For Bulk SMS Sending Can Help Your Business Grow
-
3D Technology2 years ago
Mobile 3D Scanners: Revolutionizing 3D Scanning Technology
-
3D Technology2 years ago
3D scanning technologies and scanning process
-
Business Solutions1 year ago
Understanding A2P Messaging and the Bulk SMS Business Landscape
-
Business Solutions1 year ago
The Power of Smarts SMS and Single Platform Chat Messaging
-
Automotive2 years ago
DSRC vs. CV2X: A Comprehensive Comparison of V2X Communication Technologies