Designing and training your own custom neural network can be daunting, but it can be surprisingly easy with the correct tools. This tutorial will give you guidance on how to use neural chips and an AI SDK to design and train your own neural network without needing deep learning expertise.
You will need a few things to make your own neural network with neural chips and AI SDK. First, you will need a computer with a good CPU and enough RAM to handle the data processing involved in training a neural network. You will also need a sufficiently large dataset to train your neural network. Finally, you will need the AI SDK, which can be downloaded for free from many places online.
Designing the Neural Network
When designing a neural network, there are a few key things to keep in mind. First, you need to determine the number of input and output nodes. Second, you need to choose an appropriate activation function for each layer. And third, you need to select a suitable optimization algorithm.
Fortunately, there are many resources available that can help with this process. For example, the Neural Network Toolbox in MATLAB provides functions for creating and training neural networks. There are also many online tutorials that can walk you through the process step-by-step.
Once you’ve designed your neural network, it’s time to train it. This is where the AI SDK comes in. The SDK offers a number of different algorithms for training neural networks, including backpropagation and stochastic gradient descent. You can also use the SDK to monitor the training process and ensure that the network converges toward a solution.
After training is complete, you can use the neural network for inference. This means using it to make predictions on new data points. The AI SDK provides several different ways to do this, including using a trained model directly or exporting a model to ONNX format and using it in another tool such as TensorFlow or PyTorch.
Making a neural network with neural chips and AI SDK is a great way to get started with SDK deep learning. By following this tutorial, you’ll be able to design and train your own neural network in no time.
This tutorial guide will teach you how to configure the AI SDK on your machine to create a neural network. We’ll walk you through obtaining the necessary software, importing formats and data, and generating an example neural network that performs a basic task.
First, you will need to download the AI SDK from the Intel website. Once you have downloaded the SDK, you must install it on your computer.
Once the SDK is installed, you will need to open a new project in the SDK. To do this, go to File > New > Project. In the project window, select “Neural Network” as the project type.
Once you have created your new project, you need to add input data. To do this, go to Data> Add Data. In the data window, select “Input Data” as the data type. Then, click the “Add” button and select an image file from your computer. The image file should be in .png format and should be 28×28 pixels in size.
After you have added your input data, you will need to add some output data. To do this, go to Data> Add Data again. In the data window, select “Output Data” as the data type. Then, click on the “Add” button and select an image file from your computer that contains the desired output for your neural network. The output image should also be in .png format and 28×28 pixels in size..]
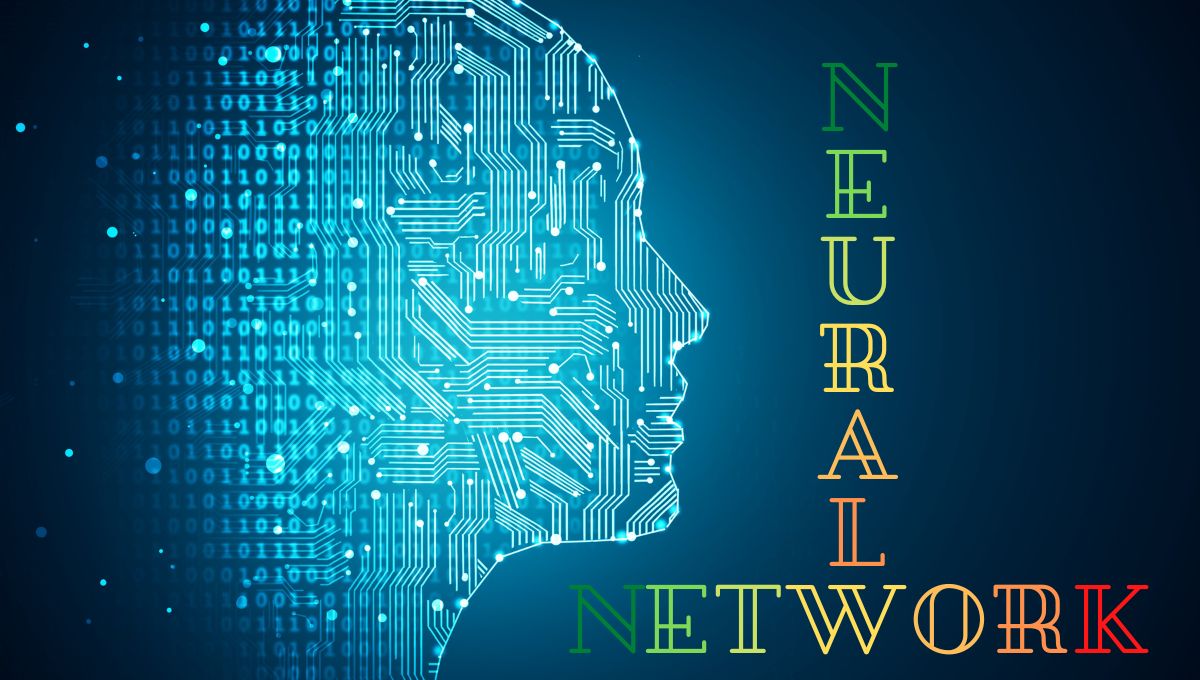
Making a Neural Network with Neural Chips
If you’re interested in creating your own neural network designs, you can do so with the help of neural chips and AI SDK.
First, you’ll need to obtain a neural chip. You can get these for sale online or at select electronics stores. Once you have your chip, install it on your computer according to the manufacturer’s instructions.
Next, download and install the AI SDK onto your computer. This software will allow you to program your neural chip with the desired functionality.
Once the AI SDK is installed, open it up and create a new project. Select “neural network” as the project type and name your project accordingly.
Now it’s time to begin coding your neural network! The first thing you’ll need to do is define the input layer. This is where you’ll specify the number of neurons in the input layer and what type of data they will be processing (e.g., images, numbers, etc.).
By defining the input layer, you’ll find it easy to determine the hidden layers. The number of neurons in each hidden layer will depend on how complicated you want your neural network design to be. For this tutorial, we’ll use two hidden layers consisting of 16 neurons each.
Finally, you’ll need to define the output layer. This is where you’ll specify the number of neurons in the output layer and the type of data they will output (e.g., classification results, probabilities, etc.).
Once you’ve defined all three layers, you can begin coding their connections. Each connection will have a weight associated with it which determines how strong the connection is. You can randomly initialize the weights or specify them yourself.
After all the connections have been coded, you’ll need to specify the activation function for each neuron. This function determines how the neuron will “fire” when it receives input from the previous layer.
There are many different activation functions to choose from, but we’ll use the sigmoid function for this tutorial.
Once all the neurons have been activated, your neural network is ready to run! You can test it on sample data to see how well it performs.
Training the Neural Network
Once you have your neural chips and AI SDK, it’s time to train your neural network. This is where the real power of neural networks comes into play. By training your neural network, you can make it smarter and more efficient at completing tasks.
There are a few different ways to train your neural network. You can use supervised learning, unsupervised learning, or reinforcement learning. Supervised learning is when you provide the neural network with input data and corresponding output data. The neural network then learns to map the inputs to the outputs. Unsupervised learning is when you provide the neural network with input data but not corresponding output data. The neural network then has to learn to find patterns in the data. Reinforcement learning is when you provide the neural network with a goal, and it has to learn how to achieve that goal.
Whatever method you choose, training your neural network will take time and effort. But it’s worth it because once your neural network is trained, it can do some amazing things!
Making a Neural Network with AI SDK
If you’re looking to make your own neural network with AI SDK, there are some things you must keep in mind. First, you’ll need to select the proper neural chips for your design. There are many different chips in the market, so finding one perfect for your needs is significant.
Once you’ve selected the correct chip, you’ll need to configure it correctly. This includes setting up the proper connection between the chip and your computer. You’ll also need to set the correct parameters for training and inference.
After configuring your chip, you can use AI SDK to train and test your neural network. AI SDK provides various tools that make developing and deploying neural networks easy. With AI SDK, you can experiment with different network architectures and hyperparameters to find the best-performing model for your data.
The Different Types of Neural Networks
There are three main types of neural networks: supervised, unsupervised, and reinforcement learning. Supervised neural networks are trained on an already established system, like images with labels identifying the objects in them. Unsupervised neural networks are trained using unlabeled data, such as images. Reinforcement learning neural networks are trained using a feedback signal, such as a reward or punishment, to learn which actions lead to the best outcomes.
If you want to begin building your own neural networks, this tutorial is for you. We’ll show you how to use neural chips and AI SDKs to create your own design. With the correct tools and a little creativity, you’ll be able to make a neural network that’s uniquely yours. So, what are you waiting for? Get started today and see what you can create!