Business Solutions
Exploring TOPS in AI and Its Impact on Industrial Automation
In an era where technology evolves at lightning speed, the intersection of artificial intelligence and industrial automation is a thrilling frontier that promises to revolutionize how industries operate. Enter TOPS—Tera Operations Per Second—a game-changing metric that’s reshaping our understanding of computational power and efficiency in AI applications. As businesses seek smarter, faster, and more efficient solutions, TOPS stands as a beacon guiding them through the complex landscape of AI-driven automation. In this blog post, we’ll dive deep into what TOPS means for industries across the globe, explore its groundbreaking implications for productivity and innovation, and uncover how it’s paving the way for a future where machines not only assist but also autonomously adapt to ever-changing environments. Buckle up as we embark on this enlightening journey into the heart of AI’s impact on industrial automation!

Published
7 months agoon
By
Marks Strand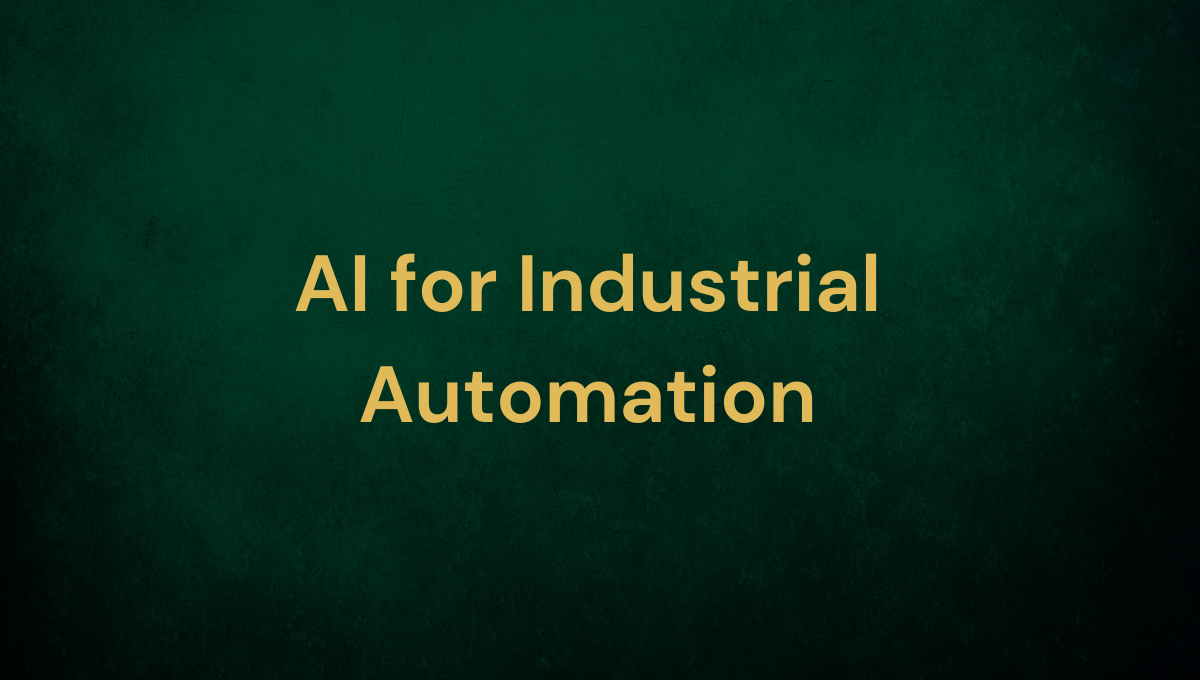
Artificial intelligence (AI) has become a cornerstone in transforming industrial automation, bringing about unprecedented levels of efficiency, accuracy, and productivity. One of the key metrics to evaluate AI performance is TOPS (Tera Operations Per Second). Understanding what is TOPS in AI and how it influences AI for industrial automation is crucial for leveraging these technologies to their full potential. This article delves into the significance of TOPS, its impact on industrial automation, and the future trends shaping this synergy.
Understanding TOPS in AI
TOPS, or Tera Operations Per Second, is a metric used to measure the processing power of AI systems. It indicates the number of trillion operations that an AI processor can perform in one second. High TOPS values are essential for handling complex computations and large datasets, which are common in AI applications. In industrial automation, where real-time data processing and decision-making are critical, having a high TOPS capability ensures that AI systems can operate efficiently and effectively.
TOPS is particularly important for tasks that require rapid processing of vast amounts of data, such as image recognition, predictive maintenance, and real-time monitoring. The higher the TOPS, the more capable the AI system is in managing these demanding tasks, leading to improved performance and outcomes in industrial settings.
The Role of AI in Industrial Automation
AI applications in industrial automation are revolutionizing how industries operate. From predictive maintenance to quality control, AI enables more efficient and accurate processes. By integrating AI, industries can automate routine tasks, reduce human error, and optimize resource allocation. AI-driven systems can analyze data in real-time, predict equipment failures, and provide actionable insights, which enhances operational efficiency and reduces downtime.
Moreover, AI enhances the flexibility of industrial automation systems, allowing them to adapt to changing conditions and demands. This adaptability is crucial for industries that require high levels of customization and precision, such as automotive manufacturing and pharmaceuticals. By leveraging AI, these industries can achieve higher productivity and maintain competitive advantages in their respective markets.
How TOPS Enhances AI Performance
TOPS is a critical measure of AI performance because it directly impacts the processing speed and efficiency of AI algorithms. High TOPS values enable AI systems to perform complex calculations quickly, which is essential for real-time applications. In industrial automation, this means that AI can process sensor data, control machinery, and make decisions without delays, leading to smoother and more reliable operations.
For instance, in a production line, AI systems with high TOPS can detect defects in products in real-time, allowing for immediate corrective actions. This rapid response helps in maintaining product quality and reducing waste. Additionally, high TOPS capabilities support advanced machine learning models that can predict maintenance needs, optimize production schedules, and improve overall system performance.
Key AI Technologies Utilizing High TOPS
Several AI technologies benefit significantly from high TOPS, particularly those used in industrial automation. Machine learning and deep learning algorithms, which require extensive computational power, perform better with high-TOPS processors. These algorithms are used for tasks such as predictive maintenance, quality control, and robotics.
For example, convolutional neural networks (CNNs) used in image recognition applications require high TOPS to process images quickly and accurately. In industrial automation, CNNs can be used to inspect products on a production line, identifying defects or deviations from the norm. Similarly, recurrent neural networks (RNNs) used in predictive analytics rely on high TOPS to analyze time-series data and forecast equipment failures.
Challenges of Implementing High-TOPS AI in Industrial Automation
Implementing high-TOPS AI in industrial automation comes with its challenges. Technical challenges include the need for robust infrastructure to support high computational power and ensuring compatibility with existing systems. Additionally, the cost of high-TOPS AI processors can be a barrier for some industries.
Logistical challenges involve integrating AI into existing workflows without disrupting operations. This requires careful planning and a clear understanding of the specific needs of the industry. Training personnel to operate and maintain high-TOPS AI systems is also crucial for successful implementation.
Solutions to these challenges include investing in scalable infrastructure, adopting open standards for compatibility, and providing comprehensive training programs for employees. Collaboration with AI vendors and experts can also help industries overcome these challenges and fully leverage the benefits of high-TOPS AI.
Future Trends: TOPS and AI in Industrial Automation
The future of TOPS and AI in industrial automation is promising, with several emerging trends poised to enhance their impact. One such trend is the development of AI processors specifically designed for industrial applications. These processors will offer even higher TOPS, optimized for the unique demands of industrial environments.
Another trend is the integration of AI with edge computing, which brings processing power closer to the data source. This reduces latency and enhances real-time decision-making capabilities. Additionally, advancements in machine learning algorithms will enable more efficient use of TOPS, making AI systems even more powerful and effective.
Predictions for the future include widespread adoption of AI-driven autonomous systems in industrial automation. These systems will rely on high-TOPS processors to perform complex tasks with minimal human intervention. The continuous improvement of AI and TOPS technology will drive innovation and growth in the industrial sector, leading to smarter, more efficient operations.
Comparing TOPS with Other AI Performance Metrics
While TOPS is a crucial metric for evaluating AI performance, other metrics such as FLOPS (Floating Point Operations Per Second) and MACs (Multiply-Accumulate Operations Per Second) are also used. FLOPS measures the computational speed of AI processors, while MACs assess the efficiency of specific operations within AI algorithms.
Each metric has its advantages and limitations. TOPS is particularly useful for applications requiring high-speed data processing, such as real-time monitoring and control. FLOPS is often used in scientific computing and research, where precision and accuracy are paramount. MACs are valuable for evaluating the performance of specific AI models and algorithms.
Comparing these metrics helps industries choose the right AI processors for their specific needs. High-TOPS processors are ideal for industrial automation applications that require rapid data processing and real-time decision-making. By understanding the strengths and limitations of each metric, industries can make informed decisions about AI adoption and implementation.
Leveraging TOPS for Real-Time Decision Making
Real-time data processing is crucial for industrial automation, where timely and accurate decisions can significantly impact efficiency and safety. High-TOPS AI systems excel in real-time applications, enabling faster and more precise decision-making.
For example, in a chemical plant, high-TOPS AI can monitor and control production processes in real-time, ensuring optimal conditions and preventing hazardous situations. The AI system can process data from sensors, detect anomalies, and adjust parameters immediately, enhancing safety and productivity.
By leveraging high-TOPS AI, industries can achieve better outcomes in real-time applications, improving overall operational performance. The ability to process data quickly and make informed decisions in real-time is a significant advantage of high-TOPS AI systems.
Ethical Considerations and Security in High-TOPS AI Systems
As with any advanced technology, deploying high-TOPS AI systems raises ethical and security concerns. Ensuring the ethical use of AI involves addressing issues such as data privacy, bias in AI algorithms, and the potential impact on employment.
High-TOPS AI systems must be designed and implemented with robust security measures to protect against cyber threats. This includes encryption of data, regular security audits, and the use of secure communication protocols. Ensuring the integrity and confidentiality of data is paramount in industrial automation, where breaches can have severe consequences.
Ethical considerations also involve transparency in AI decision-making processes and accountability for AI-driven actions. Industries must ensure that AI systems are fair, unbiased, and used responsibly. Implementing ethical guidelines and best practices can help mitigate risks and build trust in high-TOPS AI systems.
Conclusion
The integration of high-TOPS AI systems in industrial automation is transforming the industry, offering numerous benefits in terms of efficiency, safety, and productivity. Understanding what TOPS is in AI and how it impacts industrial automation is crucial for leveraging these technologies to their full potential.
The future of AI and TOPS in industrial automation is bright, with emerging trends and advancements promising to further revolutionize the sector. By adopting high-TOPS AI technologies, industries can achieve higher levels of operational performance and innovation. Embracing these technologies will drive the future of industrial operations, leading to smarter, more responsive systems that enhance productivity and sustainability. As we move forward, it is essential to balance technological advancements with ethical considerations and security measures to fully realize the benefits of high-TOPS AI in industrial automation.
FAQs for TOPS in AI and Industrial Automation
- What is TOPS in AI?
TOPS, or Tera Operations Per Second, is a metric used to measure the processing power of AI systems. It indicates the number of trillion operations that an AI processor can perform in one second, which is crucial for handling complex computations and large datasets.
- How does TOPS affect AI performance in industrial automation?
High TOPS values enhance AI performance by enabling faster and more efficient data processing. This is essential for real-time applications in industrial automation, such as real-time monitoring, predictive maintenance, and quality control, where rapid processing and decision-making are critical.
- What are the benefits of integrating AI in industrial automation?
Integrating AI in industrial automation improves efficiency, accuracy, and productivity. AI enables automation of routine tasks, reduces human error, optimizes resource allocation, and provides real-time insights, which enhances overall operational performance.
- Which AI technologies utilize high TOPS?
AI technologies such as machine learning, deep learning, convolutional neural networks (CNNs), and recurrent neural networks (RNNs) benefit significantly from high TOPS. These technologies are used in applications like predictive maintenance, quality control, and robotics in industrial automation.
- Can you provide an example of high-TOPS AI in manufacturing?
A leading automotive company integrated high-TOPS AI processors into its production lines to enhance quality control and predictive maintenance. The AI system analyzed images of car parts in real-time, detecting defects with high accuracy, resulting in a 30% reduction in defective products and decreased downtime.
- What challenges are associated with implementing high-TOPS AI in industrial automation?
Challenges include the need for robust infrastructure, ensuring system compatibility, high costs of AI processors, integrating AI into existing workflows, and training personnel to manage and maintain the technology. Solutions include scalable infrastructure, open standards, and comprehensive training programs.
- What are the future trends for TOPS and AI in industrial automation?
Future trends include the development of AI processors specifically designed for industrial applications, integration of AI with edge computing, and advancements in machine learning algorithms. These trends promise enhanced real-time decision-making, increased efficiency, and the adoption of autonomous systems.
You may like
Business Solutions
Putting Security to the Test: Exploring Automotive Penetration Testing
With the rise of connected cars, automotive penetration testing has become a vital tool in safeguarding vehicles against cyber threats. This advanced security measure ensures that your car’s systems stay resilient against potential attacks, protecting both safety and privacy. Curious about how this process secures modern vehicles? Read on to explore the cutting-edge world of automotive cybersecurity.

Published
21 hours agoon
February 21, 2025By
Adva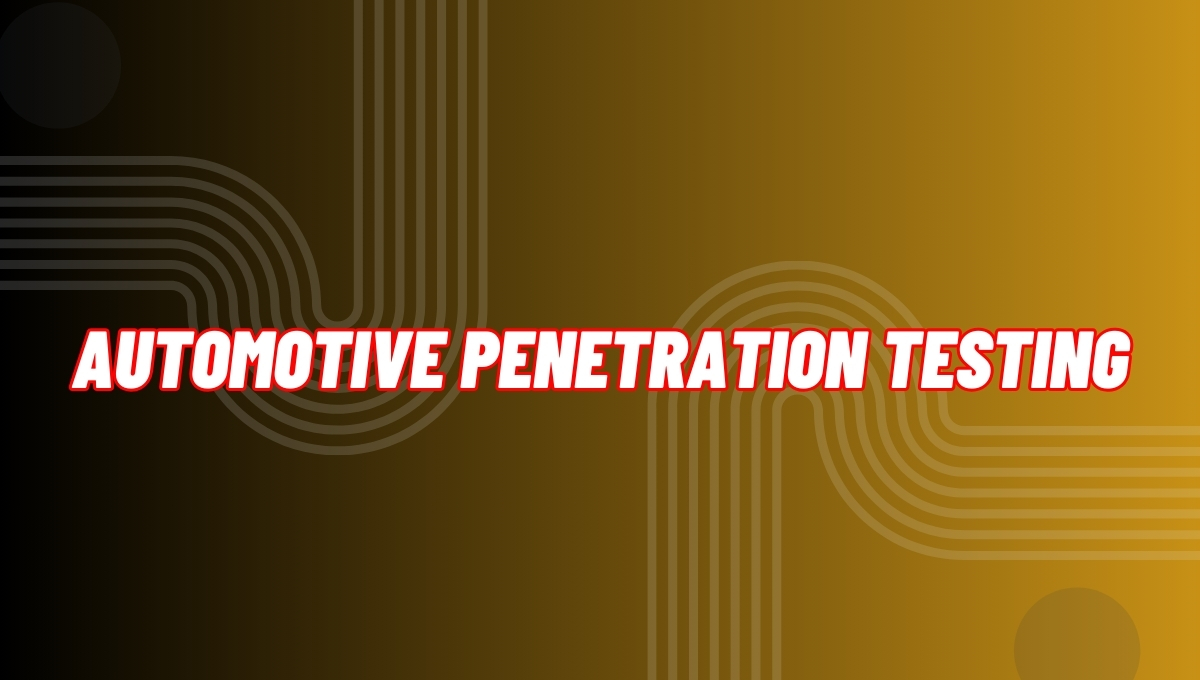
Modern vehicles are complex systems, increasingly reliant on software and connectivity. This technological evolution, while offering numerous benefits, has also introduced potential cybersecurity vulnerabilities. To proactively identify and address these weaknesses, automotive penetration testing, or “pen testing,” has become a crucial practice. This article explores the world of automotive pen testing, examining its importance, methodologies, and the challenges involved.
Automotive pentesting is a simulated cyberattack conducted on a vehicle’s systems to identify and exploit vulnerabilities before malicious actors can. It’s a proactive approach to security, mimicking real-world attack scenarios to assess the effectiveness of existing security measures. Unlike traditional software pen testing, automotive pen testing considers the unique complexities of vehicle systems, including their interconnectedness and real-time operational requirements.
The importance of automotive pen testing cannot be overstated. It helps:
Identify vulnerabilities: Pen testing can uncover weaknesses in the vehicle’s software, hardware, and communication protocols that could be exploited by hackers.
Assess security posture: It provides a comprehensive evaluation of the vehicle’s overall cybersecurity resilience.
Validate security controls: Pen testing verifies the effectiveness of implemented security measures, such as firewalls, intrusion detection systems, and encryption.
Improve security: By identifying and addressing vulnerabilities, pen testing helps to strengthen the vehicle’s security posture and reduce the risk of successful attacks.
Meet regulatory requirements: Increasingly, automotive cybersecurity regulations, like UNR 155, require manufacturers to conduct pen testing as part of their cybersecurity validation process.
Automotive pen testing involves a multi-faceted approach, often incorporating various methodologies:
Black box testing: The pen tester has no prior knowledge of the vehicle’s systems and attempts to find vulnerabilities from the outside.
Gray box testing: The pen tester has some knowledge of the vehicle’s systems, which can help to focus the testing efforts.
White box testing: The pen tester has full access to the vehicle’s systems, including source code and design documents. This allows for a more in-depth analysis.
Specific techniques used in automotive pen testing include:
Network scanning: Identifying open ports and services on the vehicle’s network.
Fuzzing: Sending large amounts of random data to the vehicle’s systems to identify potential crashes or vulnerabilities.
Reverse engineering: Analyzing the vehicle’s software and hardware to understand how it works and identify potential weaknesses.
Wireless attacks: Testing the security of the vehicle’s wireless communication channels, such as Bluetooth and Wi-Fi.
CAN bus manipulation: Analyzing and manipulating the Controller Area Network (CAN) bus, the primary communication network within the vehicle.
Performing effective automotive pen testing presents several challenges:
Complexity of vehicle systems: Modern vehicles have millions of lines of code and numerous interconnected systems, making it difficult to test everything comprehensively.
Real-time constraints: Many vehicle systems operate in real-time, requiring pen testing techniques that do not interfere with the vehicle’s normal operation.
Safety considerations: Pen testing must be conducted carefully to avoid causing damage to the vehicle or creating safety hazards.
Specialized expertise: Automotive pen testing requires specialized knowledge of vehicle systems, communication protocols, and cybersecurity techniques.
To overcome these challenges, automotive pen testers utilize specialized tools and techniques. These include:
CAN bus analysis tools: Software and hardware tools for analyzing and manipulating CAN bus traffic.
Automotive security testing platforms: Integrated platforms that provide a range of tools and capabilities for automotive pen testing.
Hardware-in-the-loop (HIL) testing: Simulating real-world driving conditions to test the vehicle’s security in a controlled environment.
The results of automotive pen testing are typically documented in a report that details the identified vulnerabilities, their potential impact, and recommendations for remediation. This report is used by vehicle manufacturers to improve the security of their vehicles.
Automotive pen testing is an essential part of a comprehensive cybersecurity strategy for modern vehicles. By proactively identifying and addressing vulnerabilities, pen testing helps to ensure the safety and security of drivers and passengers. As vehicles become increasingly connected and autonomous, the importance of automotive pen testing will only continue to grow. It’s a vital practice for building trust in the safety and security of our increasingly sophisticated rides.
Business Solutions
Top 5 Benefits of AI Super Resolution using Machine Learning
Published
2 days agoon
February 20, 2025By
Roze Ashley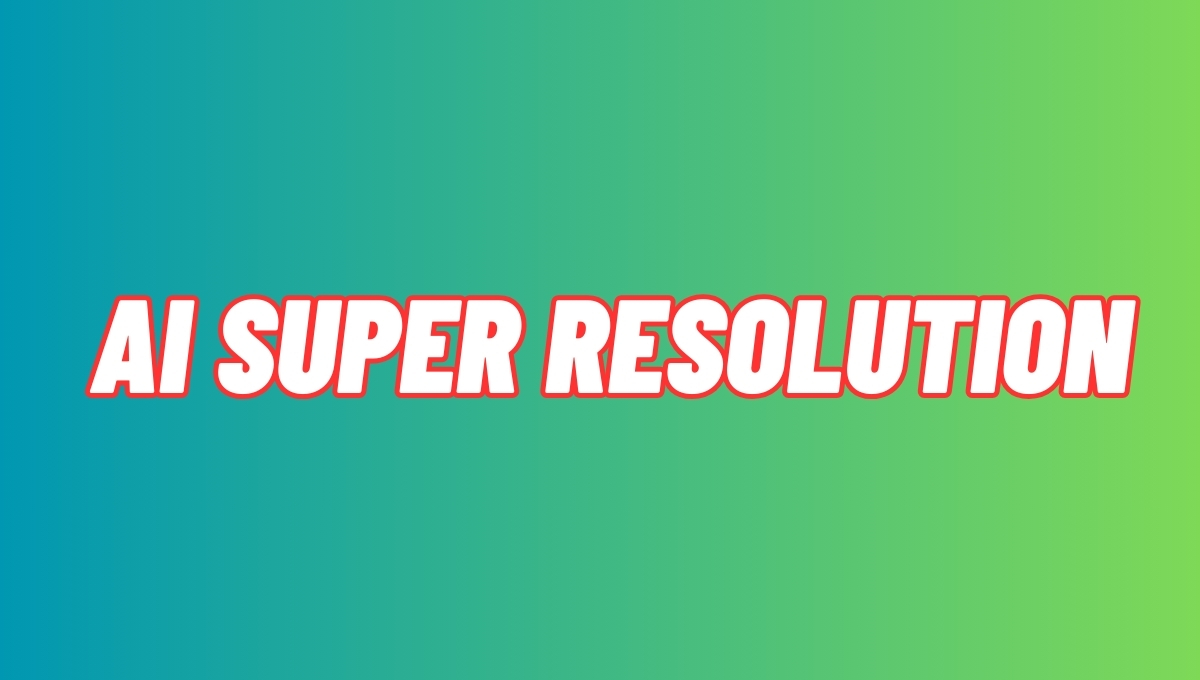
Discover how machine learning processors and AI super resolution can revolutionize your visual projects today.
At the core of visual data advancements is the machine learning processor—a purpose-built piece of hardware designed to handle the immense computations required for AI tasks. Unlike traditional CPUs or GPUs, these processors are optimized for the unique demands of machine learning models. They feature specialized circuits that accelerate matrix multiplications, handle parallel processing more efficiently, and use less power while doing so. The result? Tasks that used to take hours are now completed in seconds, allowing for real-time AI super resolution and other complex operations.
These processors are the unsung heroes of AI. They quietly process millions, sometimes billions, of calculations to ensure every pixel is rendered with precision. The combination of their advanced hardware architecture and the latest in machine learning frameworks ensures that even the most intricate details are captured, making them essential for any AI-driven application. Whether you’re working with large-scale datasets or performing edge computing tasks, machine learning processors are what keep everything running smoothly.
The Art of Clarity: AI Super Resolution in Action
AI super resolution has turned what once seemed impossible into routine. Consider a grainy photo from a decade ago, taken on an early digital camera. With traditional methods, attempting to upscale it would only result in a bigger, blurrier image. But with AI super resolution, the process is completely different. By training neural networks on countless examples of low- and high-resolution images, these systems learn to add details that weren’t visible before. They don’t just make an image larger; they reconstruct it, filling in textures, edges, and fine details in a way that looks natural.
This technology is making waves across industries. In healthcare, radiologists are using AI super resolution to sharpen MRI scans and x-rays, revealing tiny anomalies that were previously too faint to detect. In entertainment, filmmakers are restoring decades-old movies to their original glory, presenting them in 4K or even 8K quality. And in everyday applications, from security cameras to personal photography, AI super resolution is helping people see the world with a clarity that was once reserved for high-end professional equipment.
5 Ways AI Super Resolution Outshines Traditional Techniques
- Superior Detail Restoration:
Unlike traditional upscaling methods, AI super resolution doesn’t just stretch pixels; it adds new information. The resulting images look sharp, natural, and incredibly detailed. - Faster Processing Times:
Coupled with machine learning processors, AI super resolution works quickly. What used to take hours can now be done in minutes, or even seconds, depending on the hardware. - Scalability Across Resolutions:
From standard definition to ultra-high definition, AI super resolution can handle a wide range of input qualities, delivering consistent improvements regardless of starting resolution. - Application Versatility:
The technology isn’t limited to photos. It enhances videos, improves streaming quality, and even supports scientific imaging, making it a versatile tool across multiple domains. - Real-World Usability:
AI super resolution can run on edge devices, meaning it doesn’t always require a powerful data center. This makes it accessible for consumer products, smart cameras, and mobile devices.
Processing the Future
The rapid pace of innovation means that today’s machine learning processors are far more advanced than their predecessors from just a few years ago. These processors now incorporate advanced cooling systems to maintain performance under heavy loads. They use smaller, more efficient transistors that allow for higher processing speeds without increasing power consumption. And perhaps most excitingly, they are becoming more affordable, making high-performance AI accessible to smaller companies and individual creators.
As machine learning processors evolve, their impact extends beyond just image processing. They are enabling breakthroughs in natural language processing, autonomous vehicles, and even fundamental scientific research. By handling more data in less time, these processors ensure that AI applications can continue to scale without hitting performance bottlenecks. This evolution means that the machine learning processor of the future will be faster, smarter, and more energy-efficient than ever.
Where AI Super Resolution Meets Art and Creativity
When we think of AI super resolution, it’s easy to picture security systems or medical imaging. But this technology is also making waves in the art world. Digital artists are using it to breathe new life into old works, adding detail and depth that traditional techniques could never achieve. By enhancing every brushstroke and texture, AI super resolution helps preserve the original intent of the artist while bringing it into the modern era.
Photographers and videographers are also embracing this unexpected ally. Instead of shooting in the highest resolution possible—a costly and storage-intensive process—they can shoot at a more manageable resolution and rely on AI super resolution to upscale their work without compromising quality. This not only reduces production costs but also opens up creative possibilities. The technology allows creators to focus on composition and storytelling, knowing that the final output will still meet the highest standards of visual excellence.
The Broader Implications of Machine Learning Processors
Machine learning processors are the backbone of more than just AI super resolution. They power autonomous vehicles, ensuring that cars can make split-second decisions based on real-time data. They’re at the heart of cutting-edge scientific research, analyzing massive datasets to identify patterns that would take humans decades to uncover. They even support voice assistants, translating speech into text and responding to queries in milliseconds.
The broader implications of these processors are profound. By accelerating AI workloads, they free up human talent to focus on creative and strategic tasks rather than repetitive data processing. This shift not only increases productivity but also spurs innovation across industries. As more companies adopt machine learning processors, we’re likely to see even greater advancements in AI applications, from smarter home devices to more responsive healthcare technologies.
The Power Behind the Picture
The combined force of machine learning processors and AI super resolution is changing how we see the world—literally. With the ability to transform low-quality visuals into high-definition masterpieces, these technologies are not just tools; they’re catalysts for innovation. From healthcare to entertainment, art to autonomous vehicles, the possibilities are as limitless as our imagination. The next time you look at a perfectly enhanced image or watch a crisp, clear video, remember the incredible technology working behind the scenes to make it happen.
Frequently Asked Questions
- What is a machine learning processor?
A machine learning processor is a specialized chip designed to accelerate AI and machine learning workloads. - How does AI super resolution work?
AI super resolution uses advanced algorithms to enhance low-resolution images, adding detail and clarity that wasn’t there before. - Why are machine learning processors important for AI applications?
These processors provide the speed and efficiency required to handle complex calculations, making AI processes faster and more reliable. - What industries benefit from AI super resolution?
Industries such as healthcare, entertainment, security, and scientific research all leverage AI super resolution to improve imaging and analysis. - Can AI super resolution be used in real-time applications?
Yes, with the help of machine learning processors, AI super resolution can deliver real-time enhancements to videos and live streams. - What features should I look for in a machine learning processor?
Key features include energy efficiency, high processing speeds, compatibility with major AI frameworks, and scalability for various applications. - How does AI super resolution improve old photos and videos?
By analyzing patterns in low-quality media, AI super resolution fills in missing details and sharpens edges, effectively rejuvenating older content.
Business Solutions
Battlefield Situational Awareness: The Evolving Symbiosis of Technology and Tactics
Published
3 days agoon
February 19, 2025By
Roze Ashley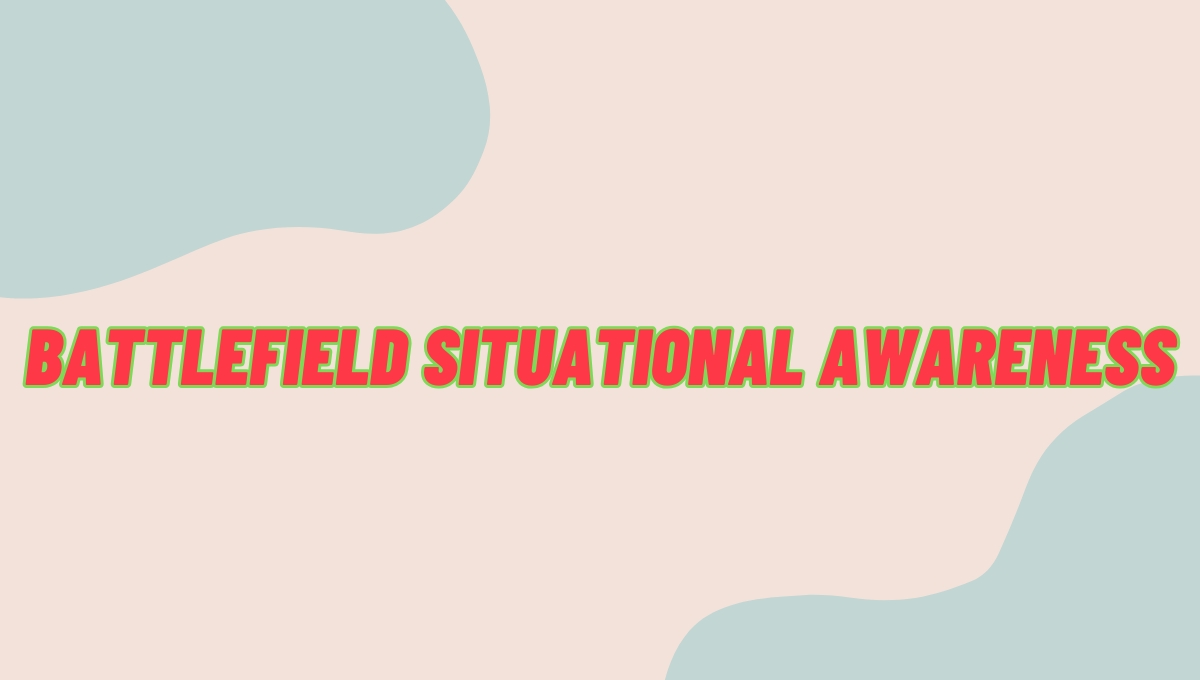
Battlefield situational awareness (SA) – the understanding of the operational environment – is the cornerstone of effective military tactics. From ancient battlefields to modern theaters of war, commanders have strived to gain a clear picture of the terrain, enemy forces, and friendly positions to make informed decisions. Today, the integration of cutting-edge technologies like video streaming, AI acceleration, and autonomous remote platforms (ARPs) is revolutionizing how SA is achieved and how tactics are employed.
The Evolution of Situational Awareness:
Historically, SA relied on human observation, reconnaissance patrols, and intelligence gathering. Information was often fragmented, delayed, and subject to human error. Modern technology has dramatically changed this landscape. Sensors, satellites, and communication networks provide a constant stream of data, painting a far more comprehensive picture of the battlefield. Learn more about edge computing solutions for tactical situational awareness in the military.
The Role of Video Streaming and AI Acceleration:
Real-time video streaming from various sources, including drones, ground vehicles, and even individual soldiers, provides a dynamic and immediate view of the battlespace. However, the sheer volume of video data can be overwhelming. This is where AI acceleration comes into play. Artificial intelligence algorithms can process vast amounts of video in real-time to:
Identify and Classify Targets: AI can automatically detect and classify enemy vehicles, personnel, and other objects of interest, freeing up human analysts to focus on more complex tasks.
Analyze Enemy Movements: By tracking enemy movements over time, Artificial intelligence can identify patterns and predict future actions, enabling proactive tactical adjustments.
Create 3D Maps and Models: AI can stitch together video feeds from multiple sources to create detailed 3D maps and models of the terrain, providing valuable information for planning and navigation.
Assess Battle Damage: AI can analyze post-engagement video to assess the effectiveness of attacks and identify areas that require further attention.
Autonomous Remote Platforms (ARPs) and Tactical Innovation:
ARPs, including drones and robots, extend the reach of SA and enable new tactical possibilities. Equipped with high-resolution cameras and sensors, ARPs can:
Conduct Reconnaissance in Dangerous Areas: ARPs can be deployed to gather intelligence in areas that are too risky for human soldiers.
Provide Overwatch and Support: ARPs can provide real-time situational awareness to ground troops, enabling them to react quickly to threats.
Perform Targeted Strikes: Armed ARPs can be used to engage enemy targets with precision, minimizing collateral damage.
Coordinate Swarm Attacks: Groups of interconnected ARPs can be used to overwhelm enemy defenses and achieve tactical objectives.
The Impact on Military Tactics:
The integration of video streaming, AI acceleration, and ARPs is leading to significant changes in military tactics:
Distributed Operations: Smaller, more agile units can operate across a wider area, leveraging ARPs and networked sensors to maintain SA and coordinate their actions.
Asymmetric Warfare: ARPs can be used to counter the advantages of larger, more conventional forces, leveling the playing field.
Information Warfare: Real-time video and AI-driven analysis can be used to disseminate propaganda and influence enemy decision-making.
Rapid Decision-Making: The ability to process and analyze information quickly enables commanders to make faster and more informed decisions, gaining a crucial advantage.
Challenges and Future Directions:
While the benefits are clear, several challenges remain:
Data Overload: Managing and interpreting the vast amounts of data generated by these technologies can be overwhelming.
Cybersecurity: Protecting networks and systems from cyberattacks is crucial.
Ethical Considerations: The use of AI in warfare raises ethical questions that need to be addressed.
The future of battlefield SA will likely involve even greater integration of AI, ARPs, and other advanced technologies. We can expect to see:
More sophisticated AI algorithms: These algorithms will be able to perform more complex tasks, such as predicting enemy behavior and autonomously coordinating swarms of ARPs.
Improved human-machine teaming: Humans and AI will work together seamlessly, with AI providing decision support and humans retaining ultimate control.
Enhanced communication networks: More robust and secure communication networks will be needed to support the flow of data between different systems.
Battlefield situational awareness has entered a new era. The convergence of video streaming, AI acceleration, and autonomous remote platforms is transforming military tactics and the very nature of warfare. As these technologies continue to evolve, the ability to gain and maintain SA will be more critical than ever, determining victory or defeat on the battlefields of the future.

Putting Security to the Test: Exploring Automotive Penetration Testing

Top 5 Benefits of AI Super Resolution using Machine Learning

Battlefield Situational Awareness: The Evolving Symbiosis of Technology and Tactics
Trending
-
Marketing & Analytics2 years ago
A Complete Guide To HubSpot’s New B2B Marketing, Sales Hub, and Prospecting Tool
-
3D Technology2 years ago
3D Scanner Technology for Android Phones: Unleashing New Possibilities
-
Marketing & Analytics2 years ago
How SMS Services And Software For Bulk SMS Sending Can Help Your Business Grow
-
3D Technology2 years ago
Mobile 3D Scanners: Revolutionizing 3D Scanning Technology
-
3D Technology2 years ago
3D scanning technologies and scanning process
-
Business Solutions1 year ago
Understanding A2P Messaging and the Bulk SMS Business Landscape
-
Business Solutions1 year ago
The Power of Smarts SMS and Single Platform Chat Messaging
-
Automotive2 years ago
DSRC vs. CV2X: A Comprehensive Comparison of V2X Communication Technologies